Why is structure prediction important?
Knowing the molecular conformation and the shape of a certain protein enhances our understanding of its function, biophysical properties (developability) and the potential targets that it might bind to 1–3. Traditionally, protein structure determination relies on time-consuming and expensive methods such as X-ray crystallography, cryo-electron microscopy (cryo-EM) and nuclear magnetic resonance (NMR) 4,5.
For these reasons, protein structure prediction has emerged as an interdisciplinary research field that attracted scientists from multiple disciplines, including biochemistry, statistics, physics, and computer science 6, promising to decrease the cost and time involved in experimental protein structure determination. Thus, the increasing interest in the field (Figure 1) motivated the development of in silico tools and softwares that predict protein structures starting from the simple input of their amino acid sequences.
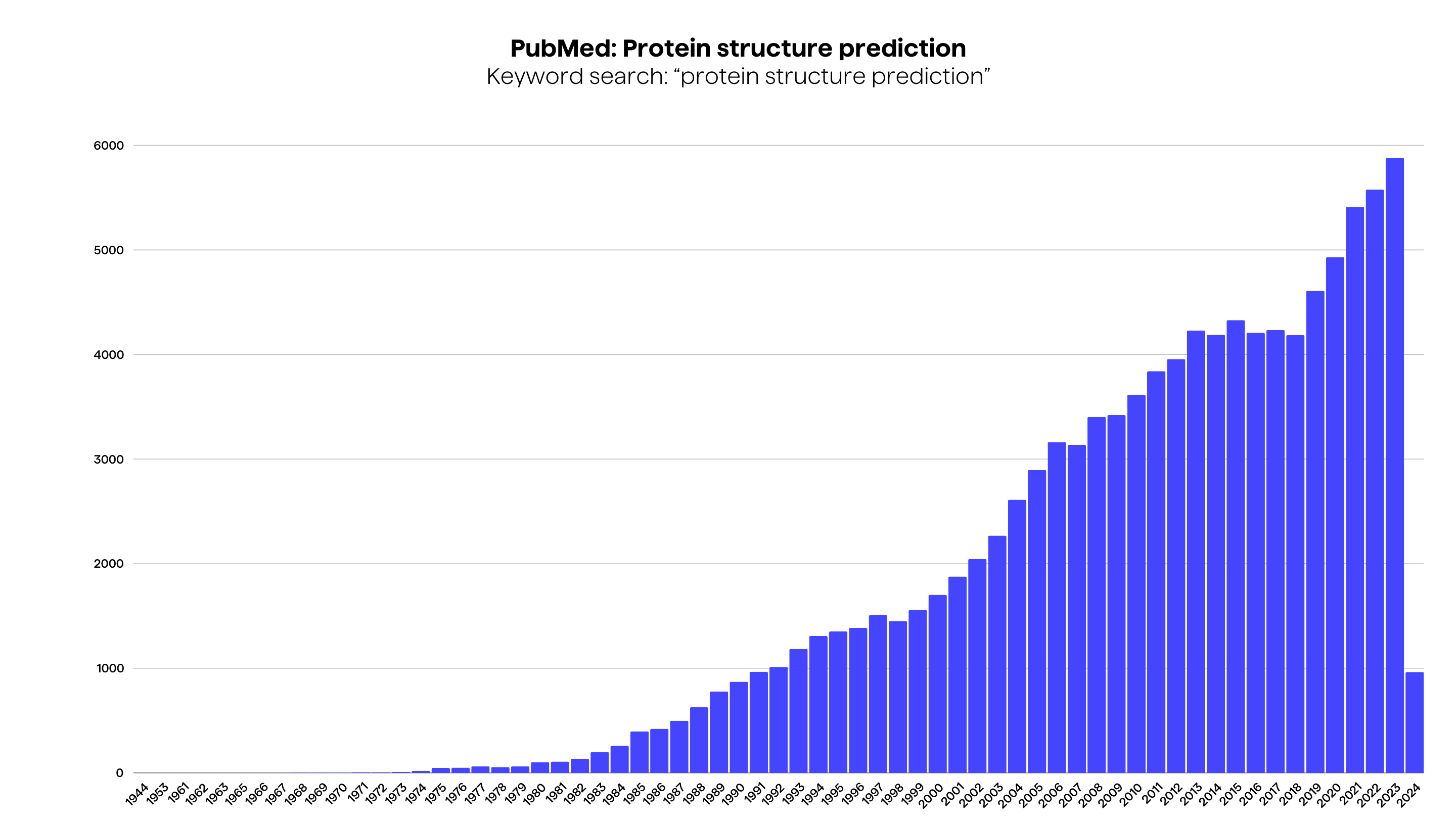
A brief history of structure prediction
Due to the increased interest in structure prediction, the field witnessed the establishment of the Protein Structure Prediction Centre 7 which conducts a community-wide experiment to assess the quality of these tools and softwares, famously known as the Critical Assessment of Methods in Protein Structure Prediction (CASP), every two years.
Among other benchmarking criteria, CASP examines the performance of newly-developed structure prediction tools by checking the atomic deviation between predicted and experimental structures (Root Mean Square Deviation, known as RMSD) on either the full protein structures or specific domain(s) 8. The lower the RMSD between the predicted and the experimental (ground truth) structures, the better the tool is.
Recently, CASP14 highlighted the drastic improvement that can be achieved on protein structure prediction accuracy when the principles of machine learning (ML) are integrated within these tools 9.
Among these tools, AlphaFold 10 and RoseTTAFold 11 have achieved impressive accuracy when trained on all the experimental structures (by their release date) in the publicly available Protein Data Bank (PDB) 12 .
Despite these advancements, the accurate prediction of antibody structures, and therefore their structure-based developability parameter values, is still considered more challenging than other types of proteins 13,14.
Why is predicting the structure of antibodies difficult?
Accurate antibody structure models are important to estimate or calculate structure-based developability parameters. There are two main reasons that make predicting antibody structures difficult:
1) The structural uniqueness of antibodies as a class of proteins
Briefly, the complete antibody molecule is formed by two identical heavy chains (H) and two identical light (L) chains, and both chains have constant ( C ) and variable (V) regions (Figure 2A) . While the constant regions of both chains are highly conserved among antibodies, and hence easier to predict their structures, the variable regions (Fv) are much more changeable in sequence which makes predicting their structures a challenging task (Figure 2B).
More specifically, and among the six CDR loops that gives each antibody molecule its unique antigen-recognition and binding properties 15, the conformation of the CDR3H has proven tricky to get right 16. This is because of its central interdomain orientation between the heavy and the light chains which plays a key role in shaping the antigen-binding domain of the antibody 17,18 (Figure 2C).
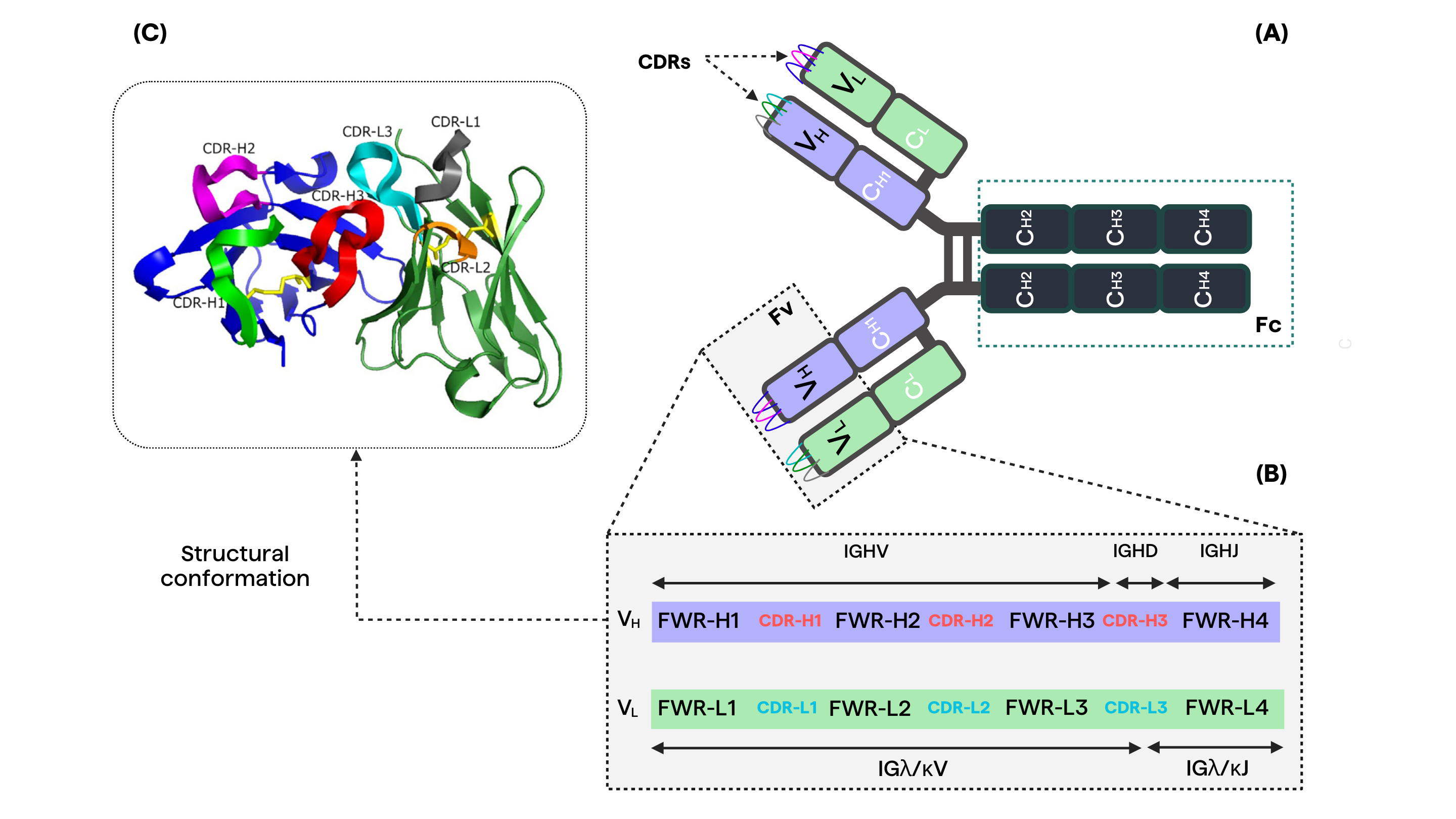
2) The bottleneck of numbers
Relatively speaking, antibodies form a tiny proportion of the general protein population with experimentally-validated structures. Speaking numbers, out of more than 200,000 structures available in the PDB (Figure 3), only around 8,000 are antibodies 21,22, forming only around 4% of the total pool of proteins in the PDB. Such a minimal ratio results in antibodies (as a class of proteins) to be under-represented in the training datasets used to develop general machine-learning based structure prediction models (like AlphaFold).
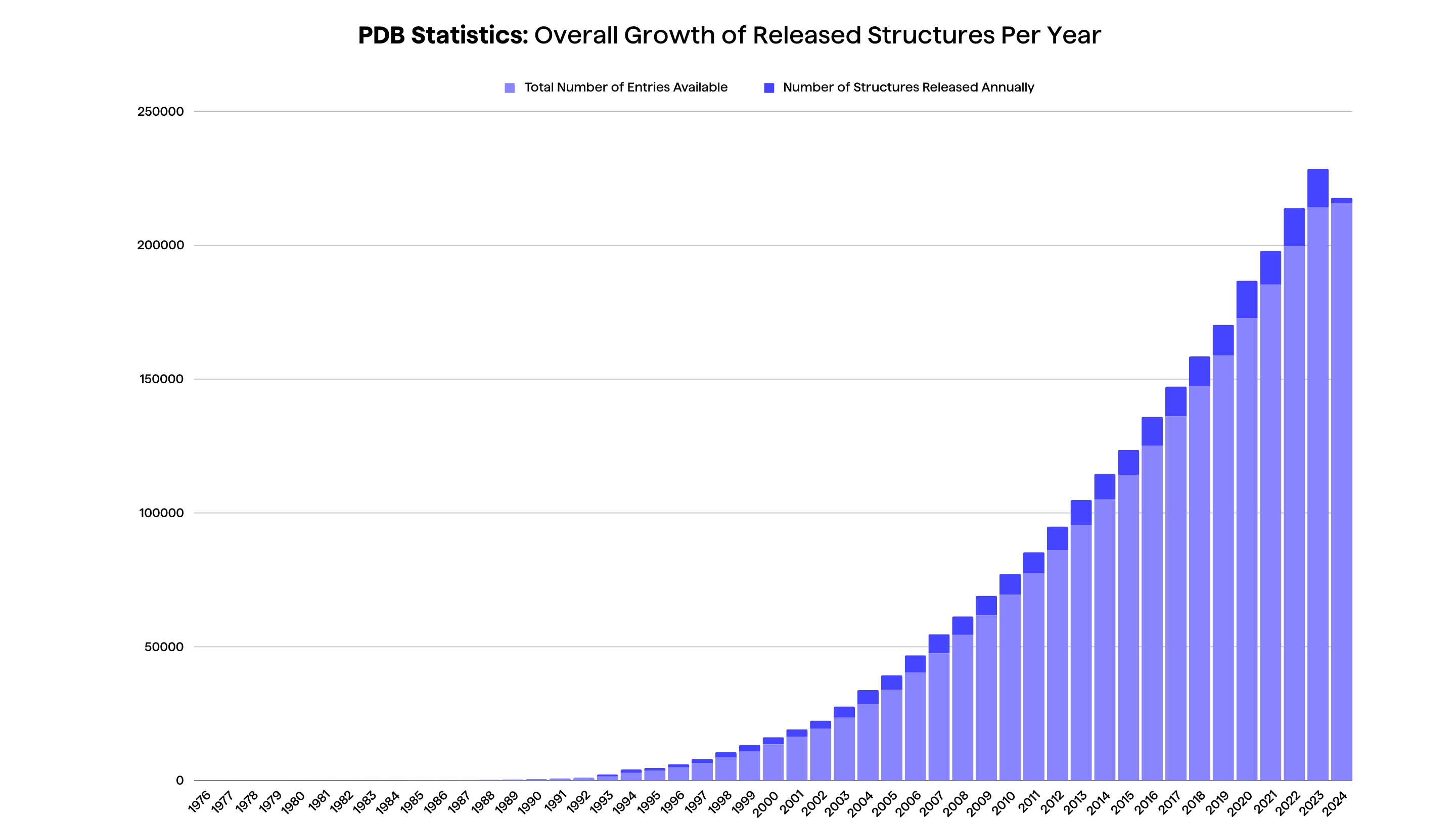
The emergence of antibody-specific structure prediction tools
To overcome the above hurdles, scientists started developing antibody-specific structure prediction tools to better capture the conformations of antibody loops1 (some of which are summarised below in Table 1).
These tools implement either 1) template-based strategy where a CDR loop with a known structure is chosen as a template structure based on its sequence similarity to the query CDR loop and grafted on the antibody structure 23,24 or 2) ML approaches, inspired by AlphaFold and its competitors 14, where the principles of artificial intelligence and knowledge obtained from training on antibody structural data are implemented to perform structure prediction.
ML-based tools (some of which summarised below) showed better performance when compared to general-purpose structure prediction methods in predicting antibody structures because they better capture the conformation of the CDR loops. For instance, ABlooper, AbFold and IgFold achieved lower RMSD measures on average, and hence better structural prediction of the CDR3H, when benchmarked against AlphaFold 1,24–26.
Figure 4 provides examples that were documented in scientific literature. It is worth noting that an updated version of AlphaFold has been announced in October 2023 and yet to be evaluated for its prediction of the CDR loop structures in antibodies.34
Recently, the OpenFold Consortium also announced two new open source structure prediction models: SoloSeq and OpenFold-Multimer. The two models (alongside the existing OpenFold tool) are designed for prediction of single proteins and protein/protein complexes.
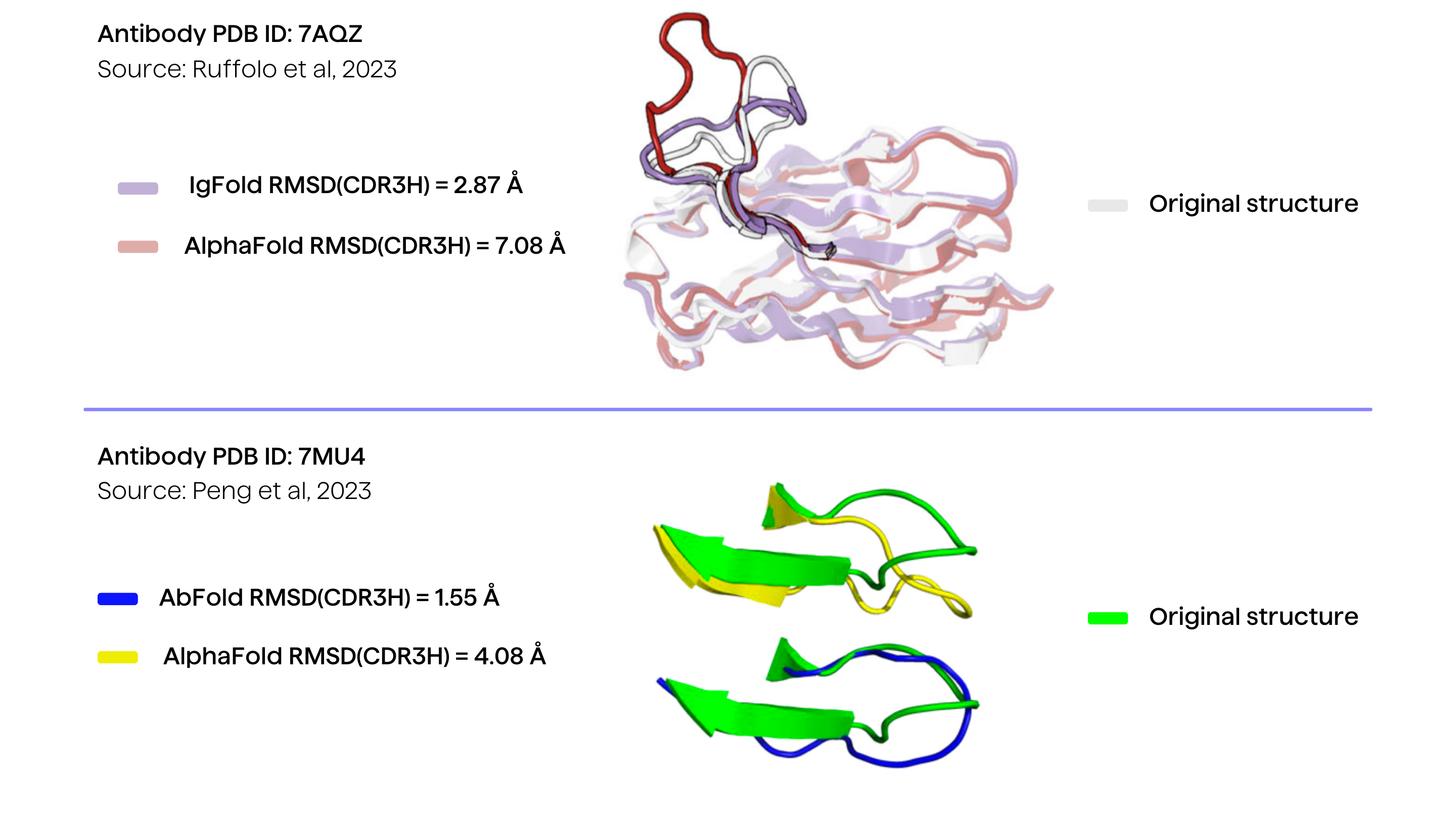
What are the limitations in regards to structural antibody developability?
Antibody prediction tools provide rigid structure output that we can use to measure structure-based developability parameters. But, several studies have shown that even with close resemblance to the ground truth structures, developability measures vary across structure prediction tools 14,27.
As antibody loops are very flexible, it is important to measure their developability on their dynamic structures rather than on their rigid ones. Indeed, only when implementing molecular dynamics (MD) to measure the developability parameters of antibodies, it is possible to reach higher agreement with developability measurements of those reported on experimental structures 26–28.
Currently, running molecular dynamics and generating developability data on the structural ensemble of antibodies are still considered lengthy and computationally-intensive procedures. However, ML-based MD may offer a high-throughput solution to this bottleneck to enhance the accuracy of computational structural antibody developability assessments 29.
Brief of summary of antibody structure prediction tools
Table 1: Summary of structure prediction tools that can be used to predict antibody structures