Developing antibody therapeutics is challenging. For a single antibody molecule to reach the clinical market, it needs to exhibit desirable qualities in two main domains: 1) target (antigen) binding and 2) developability.
While target binding generally reflects the specificity and potency of the antibody therapeutic, developability is a wider term that is commonly used to refer to several pragmatic considerations of therapeutic antibody design such as its safety, manufacturability and storability 1,2.
Overall developability is measured by quantifying several physicochemical and biophysical parameters on the antibody molecule including solubility, stability, immunogenicity, structural dynamics and aggregation (among others, Figure 1) 3,4.
This multifactorial nature of developability makes it tricky to achieve acceptable values for all developability parameters (DPs) at once while keeping the efficacy of the antibody intact.

How to quantify antibody developability?
Developability is usually assessed in the lab by measuring the values of developability parameters on antibody molecules using numerous assays 5,6. But, these assays take a long time to complete, and require plenty of resources and experienced labour, which makes them suitable to assess only a handful of antibodies at once.
For these reasons, researchers have been developing high-throughput computational tools that can predict or calculate the values of antibody developability parameters for large datasets of antibodies in silico 7.
These tools are being gradually adopted by the antibody discovery campaigns in large pharmaceutical corporations as a first screening step to narrow down the pool of antibody candidates that is carried forward for experimental developability assessment 8,9.
Computational tools for in silico antibody developability prediction
In abstract terms, antibody developability is governed by its amino acid sequence as well as the structural conformation of the antibody molecule in the 3D space. Thus, computational antibody developability tools can be broadly divided into two main categories, depending on its required input:
- Sequence-based developability prediction
- Structure-based developability prediction
Sequence-based tools
Sequence-based tools require the sole knowledge of the amino acid sequence of the antibody to predict DP values. The input for such tools is usually in the form of simple sequence strings or a FASTA file. Examples of these tools are BioPhi for immunogenicity prediction and sequence humanization 10 and SoluProt for solubility estimation 11.
Structure-based tools
Structure-based tools require the knowledge of the antibody structure. This can be either in the form of the experimentally defined (crystal) structure or the predicted model of the antibody molecule. In both, the input is usually in the form of a pdb file, but also the newer CIF format. Examples of these tools are FreeSASA for calculating solvent accessible surface areas 12 and PROPKA to calculate the molecular electrochemical properties such as charge heterogeneity and 3D-based isoelectric point 13,14.
A major limitation that faces structure-based in silico developability estimations is the current variance among structure prediction tools and the importance of incorporating molecular dynamic simulations in structural developability calculations 15–18. This point will be further expanded on in a future article from us on the topic.
It is worth highlighting that developability screening tools are becoming increasingly accessible not only to bioinformaticians, but also to scientists with less experience in data science or bioinformatics. Some of these tools offer user-friendly interfaces via web servers such as the therapeutic antibody profiler (TAP) 17 for the assessment of overall developability in relation to clinical stage antibodies, and CamSol for solubility prediction and sequence optimisation 19.
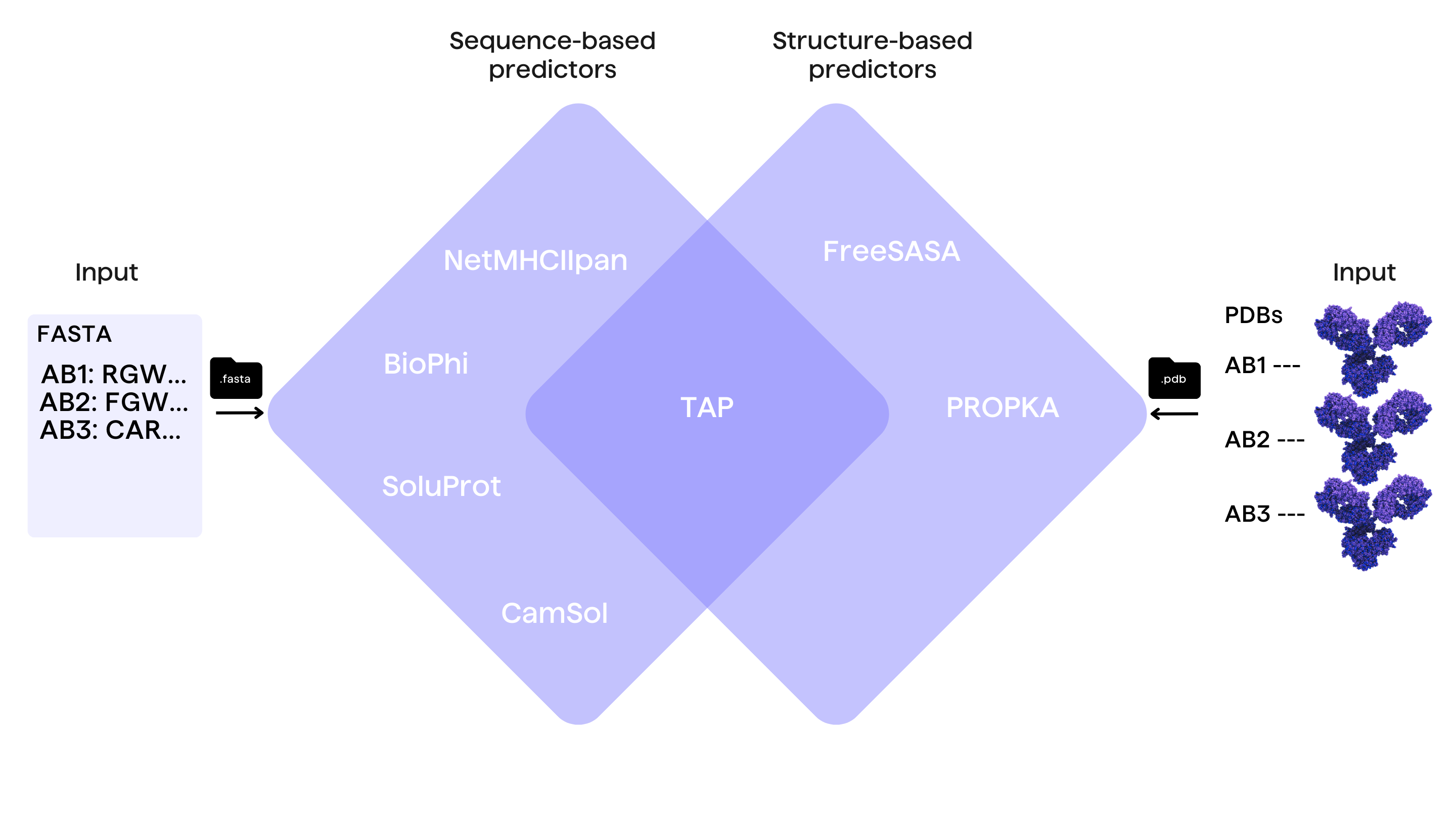
The role of machine learning in antibody developability prediction
Some developability estimation tools are based on relatively simple computation such as the instability index 20 and the sequence-based charge calculation 21, where a few data inputs is sufficient to calculate or estimate the DP value. Such parameters have been previously referred to as low-level DPs. However, the value estimation of most developability parameters is more complex and relies on larger data inputs.
For example the solubility of an antibody molecule is associated with several factors including its full sequence charge, the content and distribution of hydrophobic amino acids, full sequence hydrophobicity, the pH of the solution and expressibility of the antibody in the desired organism (among others). These parameters have been referred to as high-level DPs. Such complexity encouraged the implementation of machine learning (ML) to develop models that can learn the underlying patterns of antibody sequences in order to predict the values of high-level DPs 22,23.
Developability literature provides comprehensive reviews of ML-aided developability prediction tools 7,22,24,25. For example, netMHCIIpan is an ML model that uses artificial neural networks (ANNs) to predict the immunogenicity of proteins, including antibodies, starting from sequence input 26. This model was trained on experimental peptide-binding measurements to MHC II molecules which majorly reflects immunogenicity. Another example is SSH2.0 which uses a support vector machine-based (SVM) ensemble model trained on experimental data from 131 antibodies, to predict hydrophobic interaction risk of antibodies using sequence input 27.
However, as experimental developability data is scarce and the number of clinically approved antibodies is considered small (few hundreds), it is challenging to generalise these ML models on new antibody candidates. Indeed training ML models on a small number of sequences could likely result in overfitting as the high diversity of the potential antibody sequence space (estimated 1013 sequences for humans) is not captured in the training process 28,29.
Thus, the need for larger training datasets to better capture the true diversity of antibody sequences motivated the search for alternative larger datasets. To solve this issue, recent efforts have shown promising results in developing generative ML models trained on much larger datasets of synthetic 30 or natural 31 antibodies.
Such models have shown the ability to generate antibodies with desirable comparable or improved developability characteristics. Such models could potentially eliminate the need for developability screening for each single parameter by ensuring that the generated antibodies harbour overall desirable developability. It is worth noting that learning the developability rules from the natural antibody repertoires is a topic that will be expanded on in a separate article.