Antibody therapeutics are hard to make. In order to bring a candidate to market, there are several ‘developability’ factors that need to be considered, more broadly this includes characteristics such as, safety, immunogenicity, solubility, specificity, stability, manufacturability, and storability.1–3
Specifically, liabilities such as post-translational modifications (PTMs),4 deamidation, oxidation and isomerization,5,6 can cause serious issues in downstream development.
To complicate matters, as all of these factors are physically linked, trying to fix one issue alone (increasing specificity) may cause another factor to change (increasing aggregation).
Solving this multivariate optimization problem is not straightforward, the non-linear associations and high dimensionality make for a uniquely challenging task. Therefore, predicting and fixing these properties (quickly and cheaply) at an early stage is critical to avoid wasting resources on failed candidates.

One issue that affects many of the factors listed above that needs to be balanced when developing biologics for clinical usage is aggregation. As part of the bioinformatics liability identification toolbox, PipeBio’s aggregation analysis pipeline has been developed based on state-of-the-art techniques which include deep learning models for predicting tertiary structure combined with per-residue aggregation scoring.
This tool can help scientists dealing with information overload de-risk their therapeutic antibody discovery workflows by rapidly analyzing antibody, nanobody or TCR sequences for aggregation prone regions.
Antibody Developability: The Challenges of Antibody Aggregation
Aggregation is a major problem in antibody developability.7 Not only is aggregation dangerous (think amyloidosis), it can impact therapeutic efficacy and immunogenicity. Furthermore, aggregation is also a practical manufacturability problem8: what good is a therapy that can’t be stored and shipped to patients?
Understandably, to obtain FDA approval for a clinical usage, it is likely that proof must be given that a newly developed antibody will not aggregate. Combined with the high costs associated with testing for antibody stability, aggregation issues can dramatically slow the rate of bringing new therapeutics to market.
There are, however, many tools which can be used to support the development of stable and effective antibody therapeutics that range from experimentation, physico-chemical computation, and machine learning.
The Science of Antibody Aggregation
Antibody aggregation occurs when it is energetically favorable for monomers of proteins to come together and interact. This is dictated by factors such as pH, temperature, isoelectric point (pI), ionic strength, protein concentration, and secondary structure.9 Additionally, surface exposed hydrophobic regions pose a real danger for aggregation, as it is not energetically favorable to have hydrophobic regions facing water.
Simply put, “the inherent hydrophobic interaction of VH and VL domains limits the stability and solubility of engineered antibodies, often causing aggregation…”.10
A similar issue occurs with single-chain variable fragments (scFv), where the hydrophobic surfaces can dissociate and interact with other hydrophobic regions causing aggregation. This further explains why the smaller Camelid nanobodies (VHH)11,12 are so appealing, as instead of the typical hydrophobic regions of VH domains, they have a hydrophilic region which does not bind light chains and increases solubility.10
However, these VHHs can still suffer from misfolding and aggregation,13 so detecting and preventing aggregation can not be ignored while developing therapeutic nanobodies either.
Detecting Aggregation and Aggregation Prone Regions - Experiment vs Computation
Methods for detecting or predicting aggregation fall into the categories of experiment or computational prediction. Though experimentation is the gold standard for determining antibody aggregation, it would be massively cost prohibitive to perform experiments on the entire antibody or nanobody space.
Though they are less accurate, computational techniques are high-throughput and can be significantly more cost effective. Computational models can be physics based and rely solely on physico-chemical properties or used machine learning algorithms where experimental data is used as inputs for training models to predict aggregation propensity.
Experimentation
There are several experimental techniques which are industry standard, such as size exclusion chromatography (SEC), hydrophobic interaction chromatography (HIC), affinity-capture self-interaction nanoparticle spectroscopy (AC-SINS) and stand-up monolayer adsorption chromatography (SMAC). SEC is the standard method for measuring protein aggregation along with HIC as a complementary tool.14
Additionally, AC-SINS is useful for measuring antibody or nanobody propensity to self-associate.15 To increase confidence, multiple experimental values can be used in tandem, such as the consensus of SMAC, SINS and HIC.16
Computation
Computational methods for predicting aggregation typically take sequence or structure data as inputs, and can be physics or ML-based. In some cases, they are designed to predict aggregation per sequence, which is particularly useful for protein developers who have a lot of data to analyze and short deadlines. Or they can be designed to predict scores at the per residue level which is more explainable.
An example of a sequence-property model is the Zyggregator method, which outputs aggregation scores per residue from sequence data inputs, such as alpha and beta-helix propensity, hydrophobicity, charge, hydrophobic patterns, gatekeeper residue, and local stability into account.17
Another example is SSH2.0 (SSH stands for SMAC, SGAC-SINS, and HIC), a sequence-ML method, which uses aggregation data to train a support vector machine (SVM) ensemble model to predict aggregation.18,19
With respect to structural-property models, which require 3-dimensional atom positions as input, a popular example is Schrodinger's BioLuminate Package (AggScore20). AggScore is based entirely on tertiary structure inputs which measure the hydrophobic and electrostatic surface patches. Alternatively, Therapeutic Antibody Profiler (TAP)21,22 compares developmental antibodies to clinical-stage therapeutic antibodies using five developability values, such as CDR length, surface charge and hydrophobicity, and structural Fv charge symmetry (SFvCSP).
Note that TAP only requires sequence data as input, as the atom positions used in TAP are generated by the deep learning structural prediction tool ABodyBuilder2.
Avoiding Aggregation
It isn’t enough to simply detect aggregation liabilities, they must be removed.
This is by no means a solved problem and a complete set of attributes determining aggregation resistance are not well mapped. However, some heuristics have been developed; for example, it has been shown that aggregation of nanobodies can be circumvented by simply adding a positive charge to the CDRs.23
Other strategies include inferring stability by combining sequence and thermostability data,24 retrofitting variables domains,25 the introduction of “artificial aggregation gatekeeper residues”,26 or “camelization” of human VH domains.10
There are also in vivo platforms for evolving aggregation resistant proteins, such as the tripartite β-lactamase enzyme assay (TPBLA), which can screen and evolve ‘manufacturable’ biopharmaceuticals as well as rank innate aggregation prone peptides.27
Computational methods can also be used to engineer antibodies. For instance, to help in the selection of possible mutations for conferring aggregation resistance, the open source Aggrescan3D (A3D) tool can be used.28
A3D calculates aggregation scores per residue for a static (or dynamic using CABSs29) structure, as well as performs what-if scenarios for point mutations (using energy minimized structures in FoldX30). The key to this calculation is estimation of the solvent accessible surface areas (SASA)31 32 where the topological character of antibodies are accounted for in aggregation scoring.
Similarly to Aggrescan3D, a software tool called SolubiS attempts to optimize stability by introducing mutations that reduce aggregation.
We have performed a thorough review of available aggregation prediction servers and have provided a summary table with links below, additionally a further review of the state-of-the-art computational techniques can be found by Navarro and Ventura.33
Bioinformatics Software with Open Access Servers
Second Generation Algorithms (post-2016)
The second generation algorithms tend to take either secondary or tertiary data into account, employ machine learning or a combination of different advanced techniques.
First Generation Algorithms (prior to 2016)
The first generation algorithms exclusively deal in sequence data, are mainly amyloid focussed and do not employ machine learning.
Other Models and Algorithms
There are also published papers and methods that boast strong predictive power, though you will need to either set them up yourself or pay a license fee.
Note there are further models not reviewed by PipeBio listed here.
Predicting Developability issues in PipeBio
Lets look at an example which demonstrates the utility of some of the tools available at PipeBio for developing therapeutic biologics:
Hydrophobicity and Aggregation Score Tracks
Let’s say you have a nanobody sequence that has shown promise with respect to selectivity for a target of interest, though it also suffers from poor solubility. To improve the sequence you perform a bio-panning assay and collect enriched sequences with increased selectivity. After panning, you have a new improved set of sequences, however, their stability and solubility are still uncertain.
Using the PipeBio toolkit, you can easily investigate the potential solubility of your selected sequences for further downstream development. The first step in the developability analysis is to upload the sequences, align and annotate them.
Then, the hydrophobic patches in different regions can be compared across the aligned sequences using the hydrophobicity track. The hydrophobicity track calculates a windowed average hydropathicity score of residue hydrophobicity (Kyte-Doolittle scale).34
In this case red is hydrophobic and blue is hydrophilic. To simplify, here are two example candidates, Seq1 and Seq2:
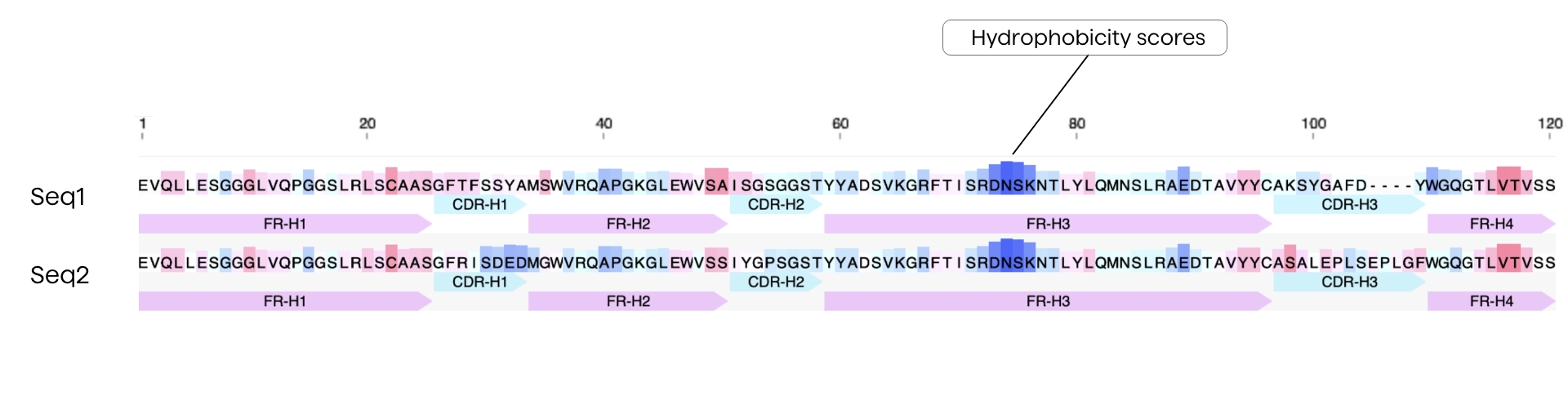
In the first iteration, based on the calculation of hydrophobicity alone it isn’t clear which of the two sequences would be more aggregation resistant.
In the CDR-H1 of Seq2 there exists a more soluble hydrophilic region, however there also appears to be a less soluble hydrophobic region in CDR-H3.
This is more clearly seen in the zoomed and cropped hydrophobicity track of the CDR-H1 and CDR-H3.
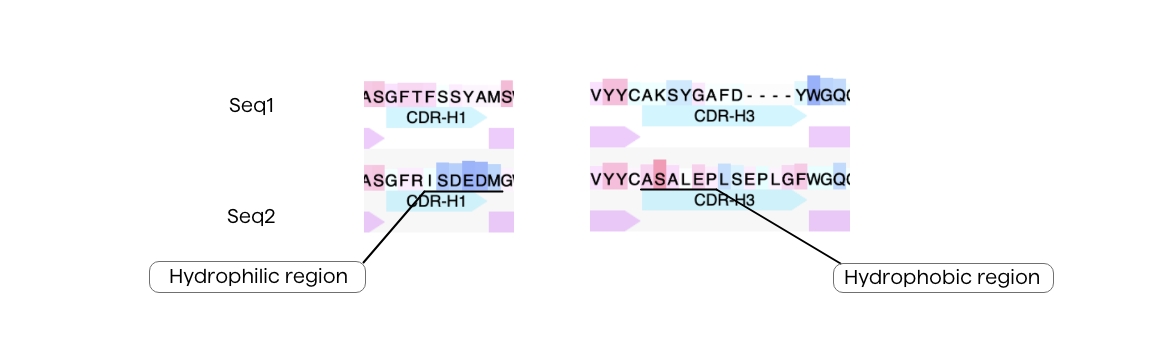
The method above does not take into account the tertiary structure of the sequences. As the nanobodies are 3-dimensional structures, what really matters are the hydrophobic regions that are exposed to the solvent.
To get a more nuanced understanding of the sequences, a method which takes into account the tertiary structure can be applied in PipeBio. First the 3D structure of a nanobody (or antibody) is predicted from sequence data using Immune Builder.21 (Alternatively, raw crystal structure data can be uploaded).
Secondly, this structure is used to calculate the per-residue aggregation score (A3D) score,35 which indicates aggregation prone patches in red and aggregation resistant patches in blue28.
Here is a zoomed and cropped view of the hydrophobicity and A3D scoring track for CDR-H1 and CDR-H3:
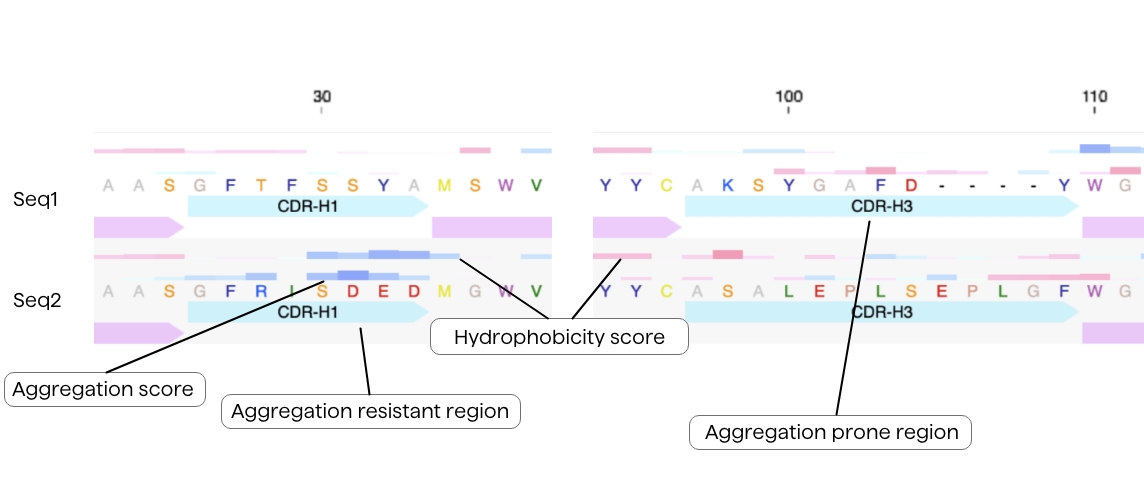
Now it is easier to see that the (blue) negative patch of A3D scores in the CDR-H1 region of Seq2 increases our confidence in the aggregation resistance of Seq2, and the (red) positive patches of A3D scores appear in the CDR-H3 region of Seq1 which decreases our confidence in the aggregation resistance of Seq1.
3-Dimensional Structural View of Aggregation Scores
Using the PipeBio protein structure display tools, a 3-dimensional surface plot of aggregation propensity scores illuminates how aggregation resistant sequences can be selected. In this case aggregation prone regions are shaded red and aggregation resistant regions are shaded blue, as shown in view of CDR-H1 (Figure 4).
Here, it is visually clear that the aggregation resistant patch in the left is smaller in Seq1 relative to Seq2, and the aggregation prone region in the right is larger in Seq1 relative to Seq2; the F at IMGT position 115 (F115), which has a high aggregation score of 1.95, is particularly prominent. This is strong evidence that indicates Seq2 will be less likely to aggregate.
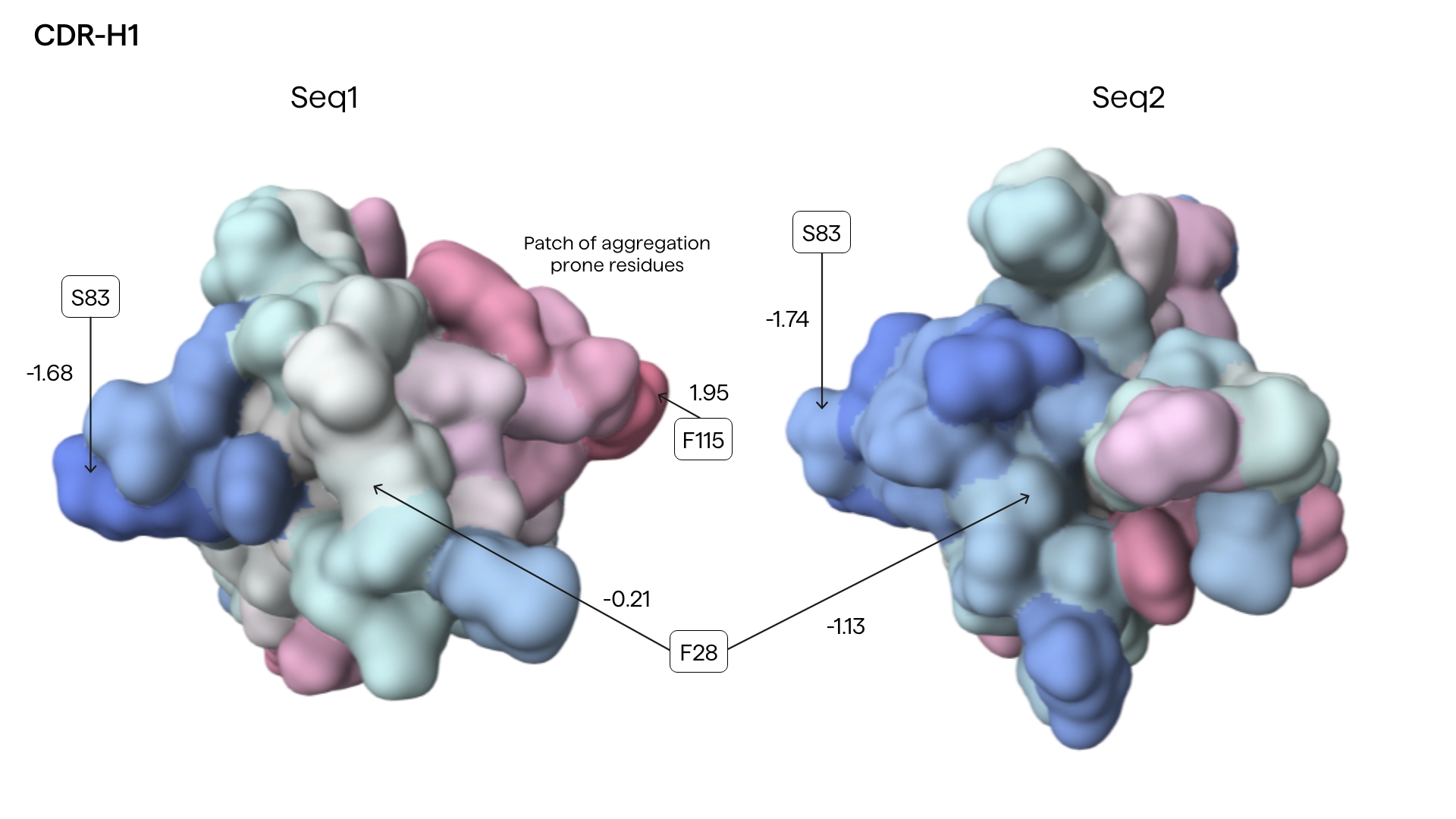
If the structures are rotated about the vertical axis by 45 degrees, the right side of the structure in Figure 4 is more easily seen – including the CDR-H3 region. In Figure 5, CDR-H3 (view 1) F115 is shown more clearly along with the surrounding surface exposed patches, such as Y108 with a score of 1.43 in Seq1.
Conversely, at IMGT 115 in Seq2, there is a leucine with a smaller score of 1.30, and the surrounding surface exposed patches are aggregation resistant with a score of -2.1 for P114; further evidence for choosing Seq2 over Seq1.
%203d%20structure%20and%20predicted%20aggregation%20propensity%20of%20nanobody.jpg)
If the structures are now rotated so the CDR-H3 regions are facing the left (Figure 6), it can be seen that the aggregation prone regions around R115 stick out in Seq1, where the same region is more concave.
Based on this conformational and residue difference, it can be seen that the aggregation score at F115 is 1.95 for Seq1 but drops to 1.30 for Seq2 (at L115).
Furthermore, the addition of an E at 113 significantly changes the aggregation scoring in that region where the E113 has a score of -1.27 and A114 has a score of 1.14.
%203d%20structure%20and%20predicted%20aggregation%20propensity%20of%20nanobody.jpg)
This theoretical example consists of real nanobody structures, namely the insoluble Dp47d (Seq1) and the soluble version HEL4 (Seq2).36
HEL4 is an isolated human VH domain with similar properties to camelid VHH domains, which has been shown to be resistant to aggregation.27 HEL4 was obtained from HEL biopanning with a phage-display library of VH dAbs - Dp47D being the template.
These nanobodies illustrate the utility of tertiary structure prediction combined with the aggregation scoring. As was clear form the first iteration, the sequentially calculated hydrophobicity was insufficient to predict the aggregation propensity of two related sequences.
Only after the tertiary structural information was added and the scores were updated to account for surface exposure was the difference in aggregation propensity more easily seen.
Conclusions
Developability characterizations for antibody discovery workflows are important as they are key to preventing downstream issues that cause delays and drive up costs. Aggregation propensity, for instance, can prevent the successful application of new therapeutics by decreasing stability and efficacy.
We have reviewed many web based tools to both predict and design structures with improved aggregation resistance.
We furthermore have integrated aggregation and 3D structure prediction tools in PipeBio which combines state of the art technology consolidated in a single platform.
Though the utility of the aggregation analysis at PipeBio is clear, some challenges (solutions still under development) still persist. Firstly, in theory the A3D scoring can take into account the dynamic nature of antibodies using software such as CABs to capture different conformations that may exist in solution.
However, this does not take into account the dynamics of the antibody when it is bound to its antigen, or correct for environmental factors such as pH. Complementary techniques will be required in the future to account for these limitations.
A second limitation is overall prediction. Though having a per-residue score is insightful, given many scientists have a large number of sequences to analyze at a time, a simple “go/no-go” score would dramatically increase throughput. This is a difficult challenge as the current state-of-the-art models, though boast strong predictive power on the training data, do not perform well on new datasets.
This brings about further challenges of handling false positives, where sequences are selected as stable when in reality will aggregate; and false negatives, where the end user loses out on potentially valuable sequences that were predicted to aggregate but would have been stable in practice.
In the future, as more experimental data is collected, deep learning models can be leveraged to help make stronger predictions that are more accurate and generalizable across domains.