UMIs and sequencing
Immune repertoire sequencing provides valuable insights into the clonal composition and diversity of the adaptive immune system1. It allows characterization of the diversity of B and T cell populations by means of sequencing antigen receptor genes of the immune cells. However, like in all sequencing applications, errors are unavoidably introduced during library preparation and sequencing which can significantly distort the outcomes true repertoire diversity.
PCR amplification steps are particularly problematic, as any errors made in early cycles get exponentially propagated in later copies. This makes it extremely difficult to differentiate true biological diversity from technical artifacts.
Unique molecular identifiers (UMIs) provide an elegant solution to overcome these limitations. By tagging each initial molecule with a unique UMI, one can group sequencing reads back to their original molecule of origin. This enables consensus-based error correction to determine the true underlying sequence.
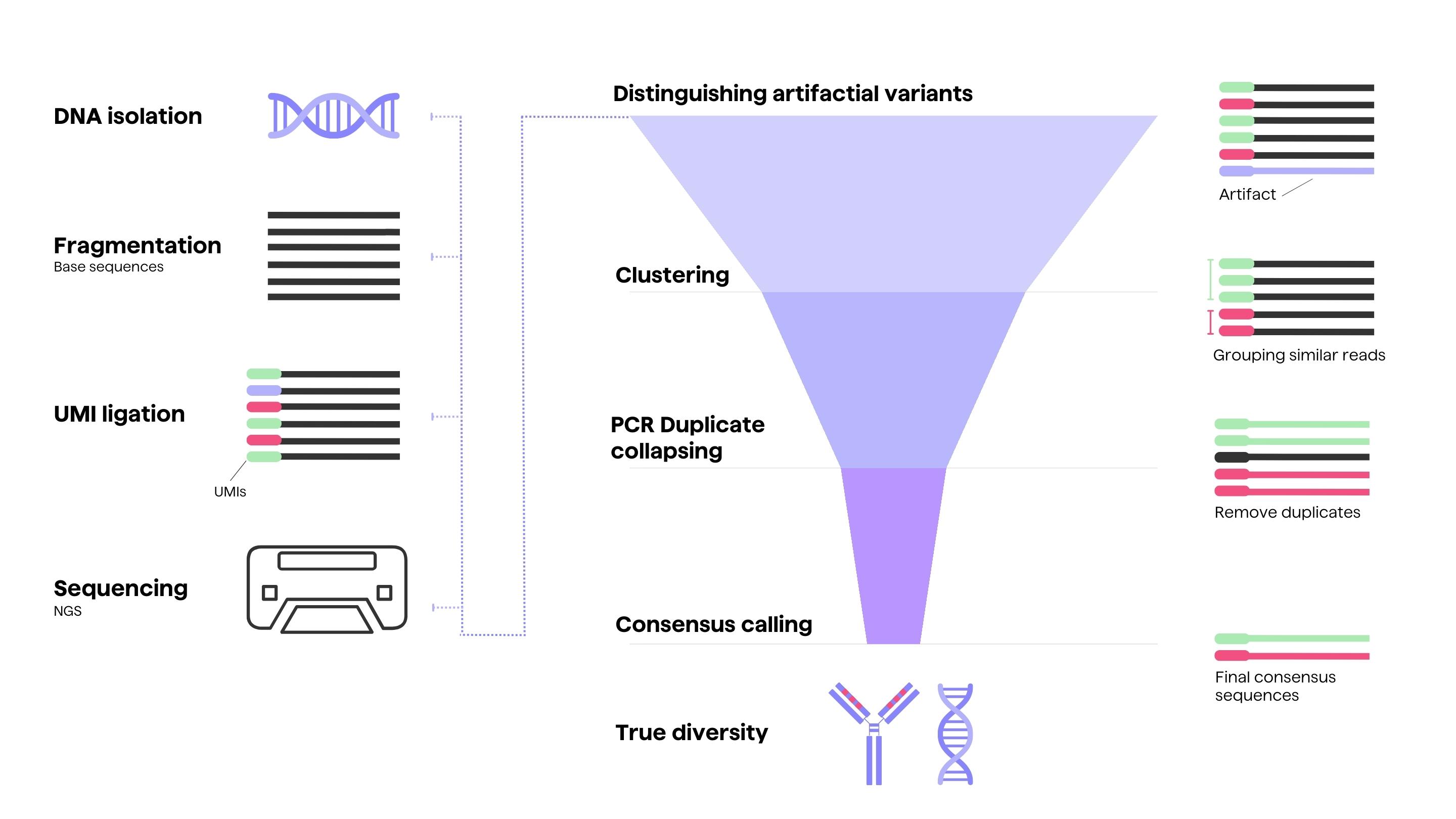
What are UMIs and how do they work?
UMIs are short, random nucleotide sequences that are attached to DNA or RNA molecules before any amplification or sequencing steps are performed. They act as unique identifiers that enable tracking of each original molecule through the entire experimental protocol.
The most common approach is tagging cDNA molecules during reverse transcription by incorporating UMIs into the primers2. Each cDNA molecule gets labeled with a UMI at one or both ends. The molecules then go through rounds of PCR amplification and finally high-throughput sequencing.
Since the UMIs act as identifiers for each initial molecule, one can computationally group reads back to their original molecule by clustering reads with identical or highly similar UMIs. This UMI-aware clustering enables consensus calling, where the consensus of all reads in a UMI group represents the true sequence of the original molecule.
This consensus approach eliminates errors introduced during amplification and sequencing, providing a more accurate picture of the actual starting molecules. This is critical for accurate diversity measurements in immune repertoire studies.
Bioinformatics tools such as UMI identification and PCR duplicate collapsing on PipeBio enable computational grouping of reads back to their originating molecules. This UMI-aware clustering mechanism empowers researchers to perform consensus calling, a process where the combined information from all reads within a UMI group yields the true sequence of the original molecule.
What's the Difference Between UMIs and Barcodes?
While UMIs and traditional barcodes may seem similar, they have distinct functions. Traditional barcodes are often used to label samples and distinguish between different experiments or subjects as highlighted by Turchaninova et al. (2016)3.
UMIs, on the other hand, are primarily used to tag individual molecules within a sample, providing a level of resolution that standard barcodes cannot achieve. In the context of single-cell sequencing, UMIs are frequently used alongside traditional barcodes to facilitate both sample and molecule-level distinctions.
UMIs for Immune Repertoire Analysis
Immune repertoire sequencing aims to capture and quantify the diversity of antigen receptor (BCR and TCR) genes present in a sample. This provides insight into the clonal composition of the adaptive immune response. However, standard molecular protocols introduce substantial errors and biases that distort the true repertoire diversity.
UMIs provide a path to accurate characterization of the lymphocyte clones4 actually present in a biological sample. By tagging and consensus calling, one can distinguish true variants from artifactual diversity generated during sequencing.
In immunology research and biologics discovery, UMIs play an important part in improving immune repertoire sequencing accuracy5:
When to Use UMIs?
UMIs are useful when PCR/sequencing errors significantly impact data reliability, and for addressing biases like false diversity and sequence overrepresentation.
Techniques and Strategies
UMI length depends on the sequencing platform and expected error rate. Longer UMIs distinguish more molecules but increase costs and complexity. Strategic UMI placement at primer 5' ends captures template and reverse transcription errors. Careful primer design enables optimal UMI use.
Sequencing Platforms and use of UMIs
Various platforms accommodate UMIs, with different library prep kits supporting UMI incorporation. Researchers should choose the optimal platform/kit for their goals and constraints.
Several experimental design choices impact UMI efficacy:
- UMI Length: Longer UMIs provide more unique identifier sequences, reducing chances of collision where different original molecules get the same UMI. But overly long UMIs reduce sequencing throughput for the gene of interest. 6-12 bp is typical.
- UMI Placement: Adding UMIs to both ends of the molecule (duplex tagging) provides the most power for error correction. But single-end tagging can suffice when sequencing length is limited.
- Primer Design: Primers can be optimized to avoid complementarity with UMI sequences, preventing bias during amplification.
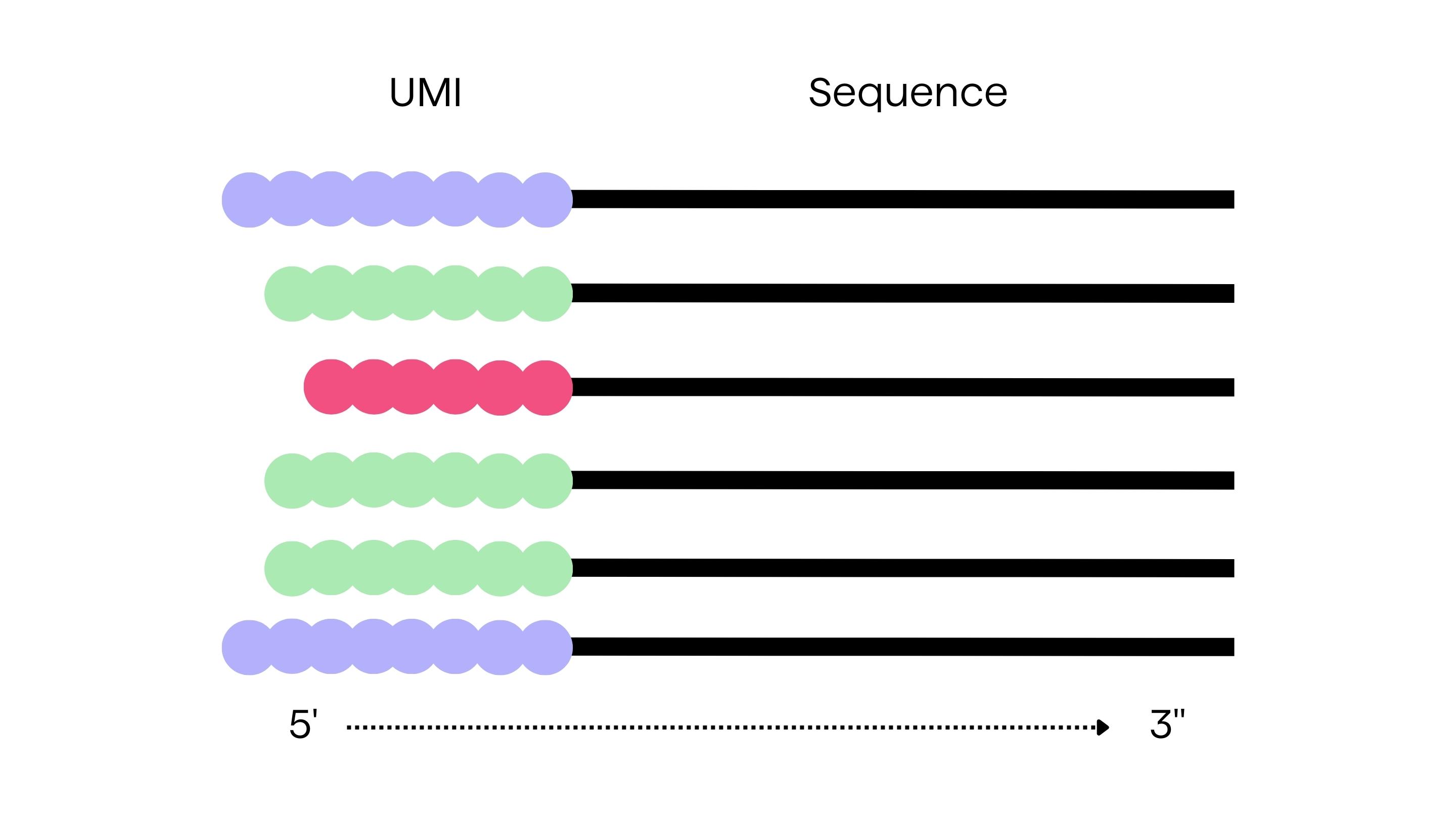
Sequencing considerations are also important:
- Platform: Illumina platforms work well, combining high throughput and substitution error profiles that UMIs help correct.
- Read Length: Long read lengths improve overlapping of paired-end reads, aiding bioinformatic UMI identification and clustering.
As illustrated by Peng et al. (2023)6 UMIs provide several key benefits for immune repertoire sequencing:
- Error Correction: Consensus calling corrects PCR and sequencing errors, revealing the true underlying sequence.
- Bias Correction: Quantification by UMI counts rather than distorted read counts normalizes for amplification bias.
- Diversity Accuracy: Error correction provides accurate measurement of true clonal diversity.
Finally, UMI-aware algorithms are needed for clustering, consensus calling, and error correction of sequencing data. This facilitates accurate downstream analysis of clonal diversity.
UMI Error Correction Algorithms
Computational approaches for UMI error correction7 include:
- UMI Clustering: Similar UMI sequences are grouped, allowing for errors.
- PCR Duplicate Collapsing: Reads with identical UMIs are collapsed.
- Consensus Calling: Within each group, the consensus sequence is determined at each position.
- UMI “Seeds” Correction: Use of UMI “seeds” that are part of the UMI sequence that is truly unique to each molecule and is used for accurate quantification and error correction in high-throughput sequencing applications. It ensures that each molecule can be distinguished from others with a high degree of confidence during data analysis. Abundant UMI "seeds" are identified, and lower abundance UMIs are error corrected by mapping to seeds.
- Bayesian Inference: Bayesian methods are used to infer the true sequence from reads with UMIs, taking into account prior information about the likelihood of errors.
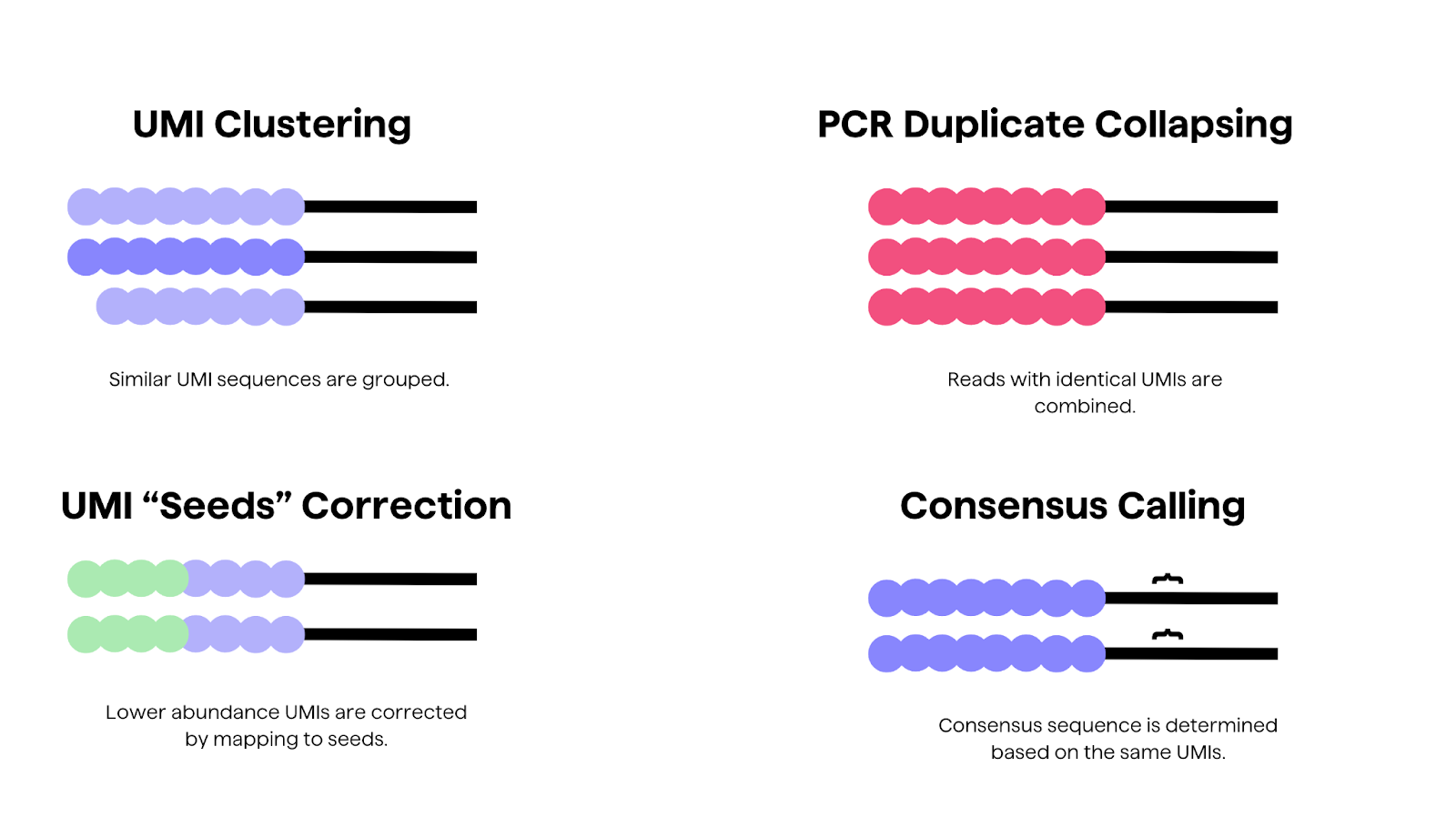
Finally, UMI counts, rather than read counts, are used for quantification to overcome amplification bias.
UMI strategy for NGS: MAF
The presence of PCR errors, including biases in Ig-seq data, still persists even when using Unique Molecular Identifiers. To address these concerns, Khan et al. (2016)5 developed a UMI strategy, known as Molecular Amplification Fingerprinting (MAF).
MAF incorporates both reverse and forward UMI tags before and during PCR, facilitating the implementation of an algorithm to rectify amplification bias. Throughout cDNA synthesis and PCR, MAF employs distinctive reverse and forward tags to meticulously monitor each individual molecule, achieving accurate quantification and bias correction.
Incorporating spike-in antibody standards, MAF has accomplished a high, 98-100% error correction rate for clonal and intraclonal variants, along with a remarkable 99% accuracy in estimating clonal frequencies. With MAF correction, predictions about immunization status based on clonal frequencies can be done in a precise manner, quantified by an “Intraclonal Diversity Index”.
How to process UMIs easily on PipeBio
On PipeBio, you can preprocess UMI-tagged sequences in a few easy steps in order to identify the origin of the sequences or distinguish true diversity in antibody and TCR sequencing.
Step 1: Identify and trim UMIs in your raw fastq files
If you have incorporated UMIs in both your forward-reverse NGS reads the tool can be run prior to merging the paired-end NGS reads in order to extract UMIs from both the forward and reverse NGS reads.
Step 2: Collapsing PCR duplicates
You can define your UMI pattern(s) both for your forward and reverse reads separately (if incorporating a similar workflow to, e.g. MAF from Khan et al. 2016).
The tool will correct probable PCR and sequencing errors by collapsing reads that have both very similar UMIs and very similar sequences. Identical or very similar reads with different UMIs (set by the error tolerance parameter) will not be collapsed. Similarly, very different reads with the same UMIs will not be collapsed.
Step 3: Outputs
You will be able to observe UMI clusters in two separate output documents:
- The collapsed consensus sequences
- Full table of sequences which allows you to see exactly which reads were grouped together for each UMI to produce the collapsed sequences
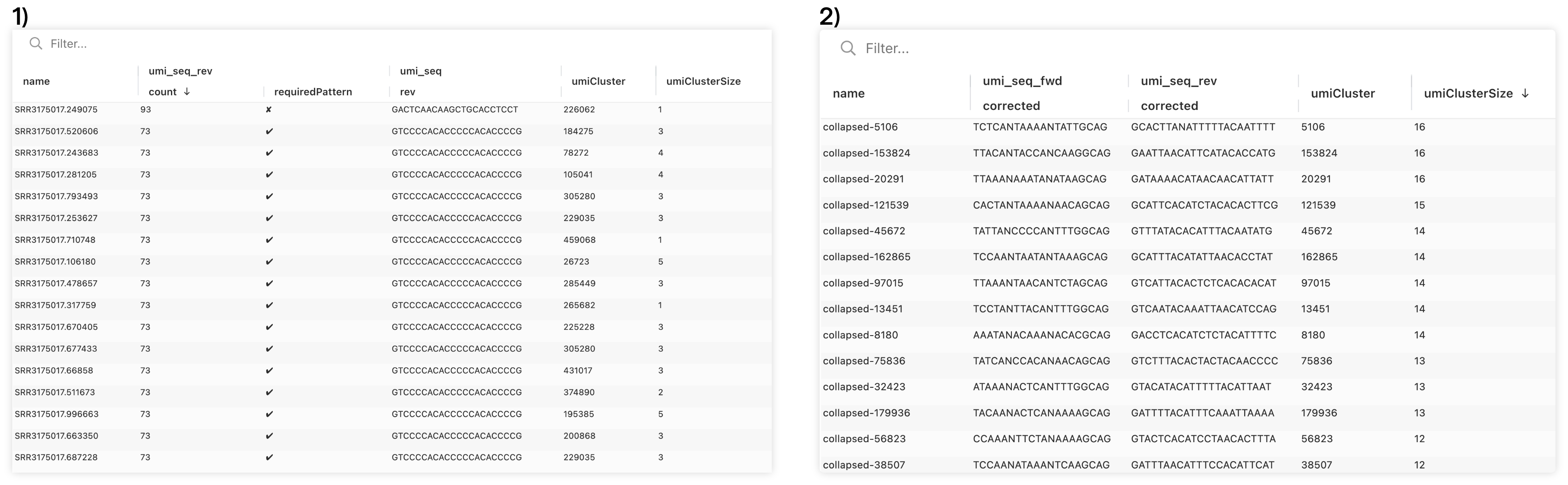
The UMI processing tools on PipeBio allow you to have control over how UMIs are collapsed, while also enabling you view summary data and to check individual UMI clusters and corresponding reads within.
Benefits and limitations of UMIs in sequencing
Studies using UMIs for immune repertoire sequencing have shown substantial improvements in quantification of diversity compared to traditional protocols. By collapsing artifactual variants, UMIs yield a more accurate picture of the true lymphocyte population.
However, limitations remain to realize the full potential of UMIs as Johansson et al. (2022)8 highlighted. Handling very low frequency clones is still difficult. Some biases like differential amplification efficiency are not fully corrected by UMIs. And error-free consensus calling requires high depth sequencing of each UMI.
Ongoing work on experimental and computational optimizations continues to expand the power of UMIs for immune sequencing. Long read sequencing technologies also show promise for improving UMI-based analysis. Despite current limitations, UMIs present a major advance towards accurate and high-resolution characterization of immune repertoires.