Introduction
The high-throughput assays enabled by multiplexing has opened up a world of interesting applications, as the technology has become a standard. We have previously given an overview of single-cell sequencing technologies in antibody research. We will analyze data from King et al. in the 2021 paper: Single-cell analysis of human B cell maturation predicts how antibody class switching shapes selection dynamics. We will provide an overview of the Chromium platform by 10x Genomics, as well as details on how to get your 10x data ready for analysis.
Index:
- Single-cell applications in immunology
- BCR repertoire analysis on PipeBio
- Appendix 1. FAQ: 10x data on PipeBio
- Appendix 2. 10x Genomics technology and Cell Ranger outputs
- References
Applications in immunology
Availability of high-throughput single-cell sequencing and RNA-seq have prompted a range of studies in BCR and TCR and repertoire profiling in humans, mice, non-human primates, including deeper analysis of B-cell maturation and class switching . Studies such one from Setliff et al. (2019) have linked single B-cells to antigen specificity by first sorting antigen-stained B-cells, then tagging both the antigen and the BCRs with the same barcode and finally creating a score for specificity from sequence counts.
A later study from the same lab by Shiakolas et al. (2021) amended the method with ligand-blocking information to both bind and block the target antigen. Both studies are summarized in a previous blog post from us. Analyzing the relationship between single-cell transcriptomics and receptor repertoires by mapping B cell or T cell clonotype functionality with BCR and TCR receptor sequencing has also garnered interest among researchers .
Another recent development in high-throughput single cell analysis potential a further enhancement to 10x Genomics’ Single Cell ATAC (Assay for Transposase Accessible Chromatin). The method, PHAGE-ATAC, developed by Fiskin et al. (2022), allows higher throughput simultaneous single cell measurements by using phage displayed nanobodies as reagents (more in our previous article).
Needless to say, single cell sequencing has much more yet to contribute to clinical therapies. Next we will dive into the details of the data that 10x Genomics libraries and NGS sequencing yields.
Preparing 10x Genomics sequence data for analysis
After library preparation and a run on the Chromium chip, the 10x barcoded and UMI-tagged samples can be sequenced by supported Illumina NovaSeq, HiSeq, NextSeq or MiSeq sequencers. During an Illumina sequencing run, the sequencers will yield base call (BCL) files, which contain per-cycle data. These files need to subsequently be converted into the more usable FASTQ file format, which contain per read data. This can be done with Illumina’s bcl2fastq conversion software or directly with cellranger mkfastq, which also uses bcl2fastq for conversion and demultiplexing. You can upload demultiplexed data or fastq files without the associated data, in case you have performed cell-sorting prior to sequencing.
A closer look at B-cell stages on PipeBio
In their 2021 paper, King et al. investigate the different stages of B-cell development in tonsils; from naive B-cells through to the affinity maturation phase in germinal centers to differentiation into memory B-cells or plasmablasts. In the study, B-cells from each stage were obtained from 9 donors, FACS-sorted and UMI-indexed with 10x Genomics Chromium and subsequently sequenced both for single-cell transcriptomics and in bulk antibody repertoires.
We decided to analyze some of this data on the PipeBio bioinformatics platform and will now take a deeper look at one of the donors’ tonsillar antibody repertoire. For this analysis, we used the unprocessed antibody repertoire data from patient BCP1, a total of 10,204,106 sequences.
After importing and merging paired-end reads, we re-annotated the data in PipeBio, which produced 7,092,132 correctly annotated reads (without frameshifts, stop codons and major liabilities).
The isotype distribution of the sequences for this patient confirms, as expected, that the sequences from naive B-cells are predominantly unswitched, mostly displaying the IgM isotype. Furthermore, the germinal center and memory B-cells contain both switched and unswitched sequences, whereas the sequences from plasmablasts are predominantly switched IgG and IgA isotypes.

We also decided to investigate patterns of isotype switching for individual antibody sequences in the dataset. First, we compared the largest clusters (clustered at 90% identical AA-sequences) to see clusters for isotype-switched sequences from naive cells to germinal centers to either plasmablasts or memory B-cells. We performed a differential enrichment analysis on clusters across samples to find statistically significant (FDR-corrected p-value >0.05) fold-changes for clusters. In the volcano plots below, we can see the fold changes between differentiation stages.
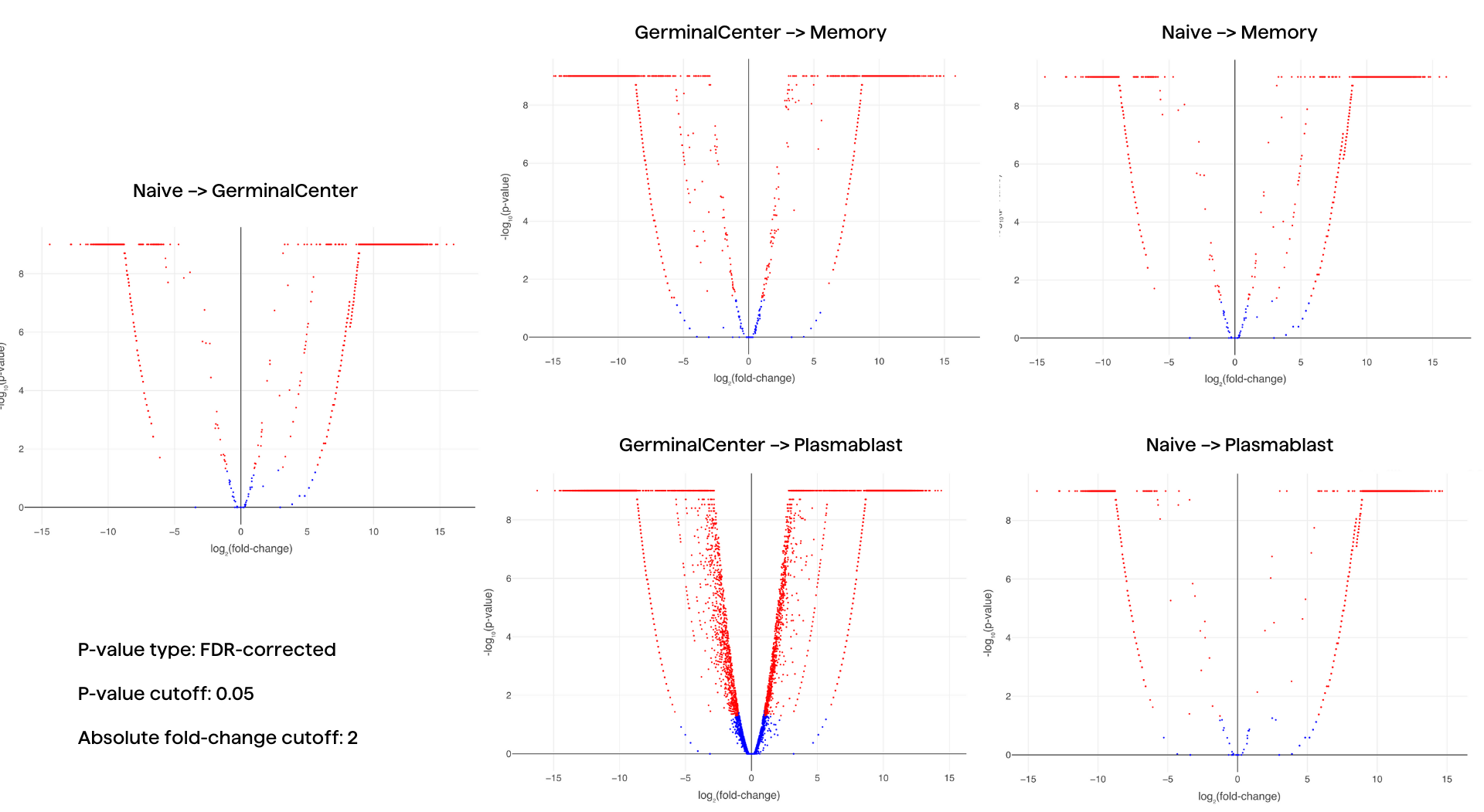
As expected, the fold changes were greatest for isotypes IgG1, IgG2, IgG3, IgA1 and specific IgM clusters when comparing germinal centers and plasmablasts. Comparing germinal centers and memory B-cells, specific IgM clusters as well as IgG1, IgG2, IgG3 and IgD had the greatest fold changes.
We also clustered the patient’s sequences at 90% identity of CDR-H3 to visualize the overall isotype landscape of this dataset. The subset ‘Total’ (B-cells FACS sorted only on CD19+, present in all cells) was excluded, since sequences from these cells did not contain information on the presumed cell stage. We identified a cluster containing multiple isotypes: IgM, IgA1 and IgA2.
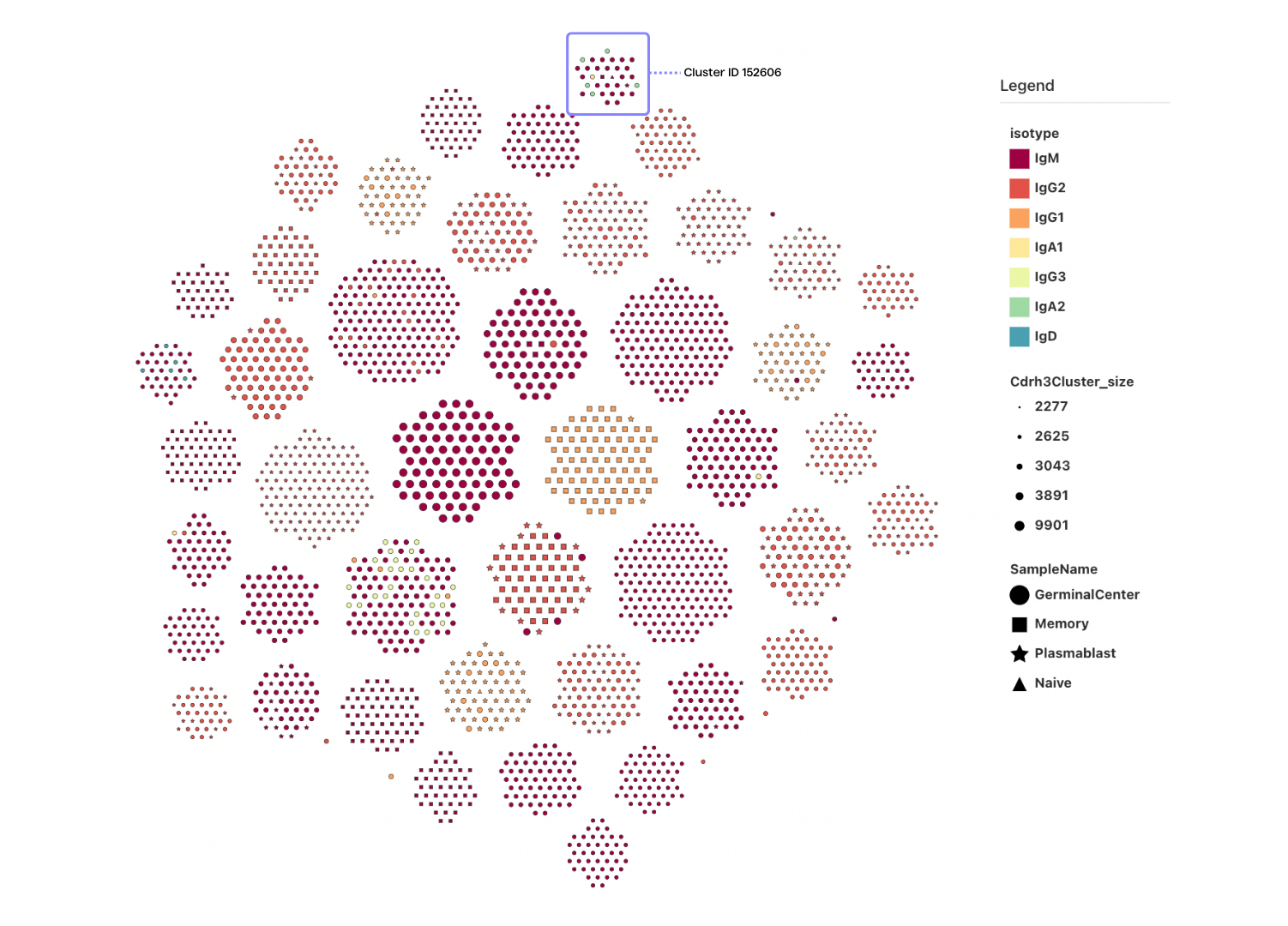
This cluster contained at least one cluster from each cell stage, denoted by the shapes of the cluster symbols. The combined cluster contained 3,113 sequences in total, predominantly of isotype IgM, indicated by the red color.
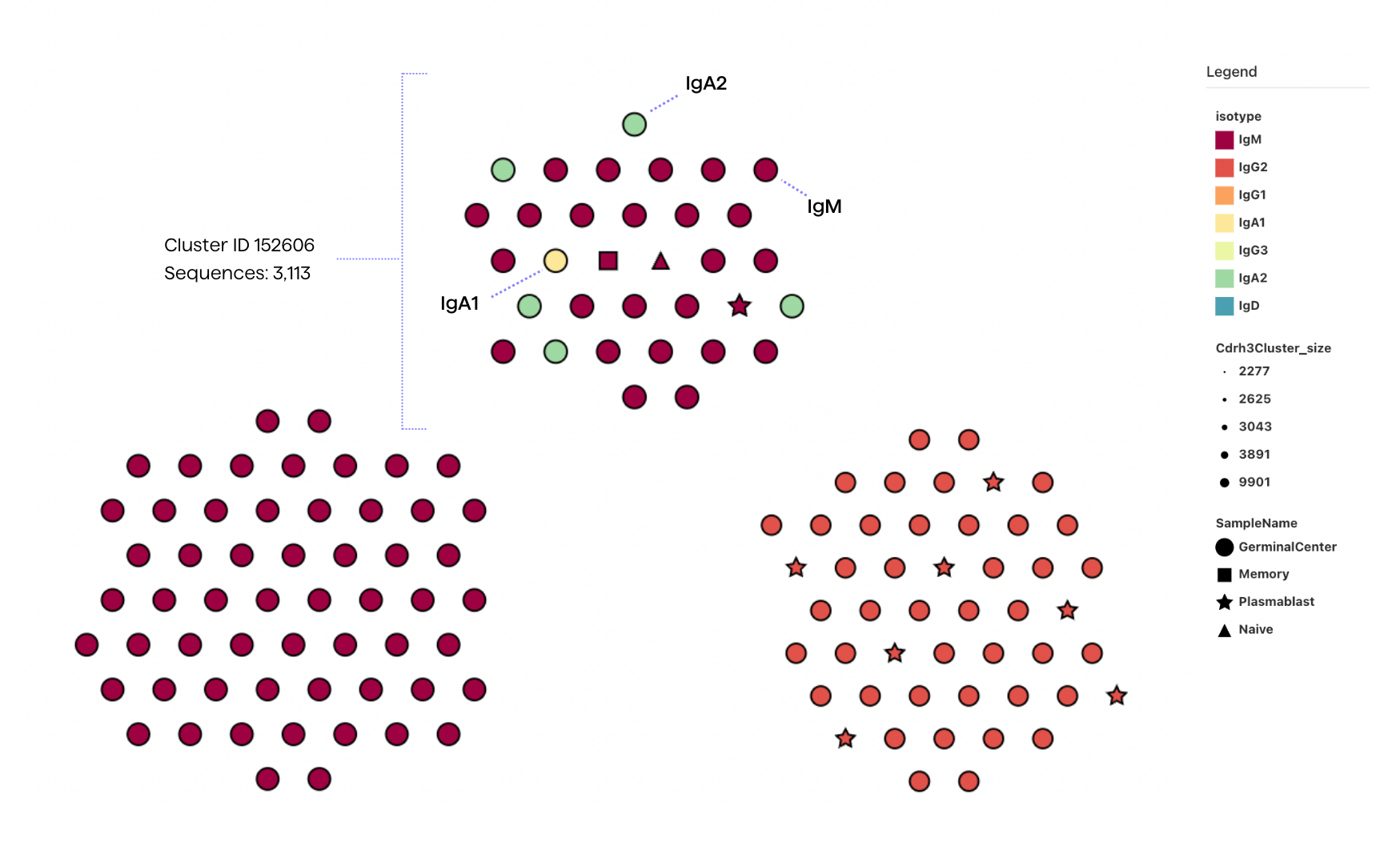
Focusing in on the subcluster, we identified and quantified the present isotypes per cell stage:
The most diverse set of isotypes were, as expected, found in the germinal center, while the other IgA1 isotypes were also found in a plasmablast sequence subcluster.
Additionally filtering the remaining 3,113 sequences by a V-gene identity of 97.5% provided us with 229 sequences and an interesting subset of both IgM and IgA1 isotypes in germinal center, memory and plasmablast B-cells.
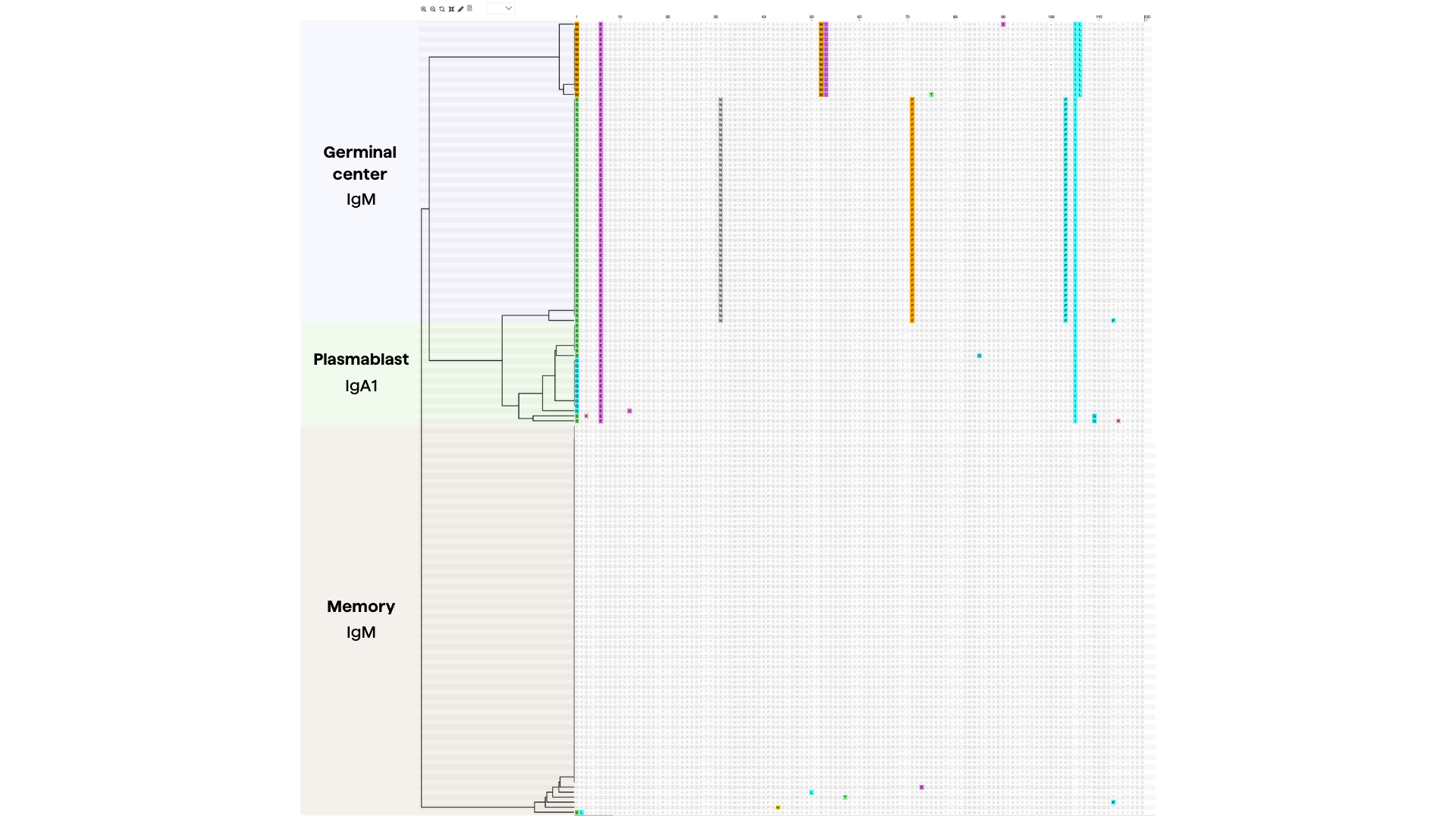
In this small subset of sequences, as expected, most diversity could be found in the class-switched IgA1, while it is actually the conserved Alanine in the CDR-H3 that determines the isotype at the conjunction of the germinal center and plasmablast in the graph.
Returning to cluster 152606, we also performed a sequence alignment on the 22 sequences (see Table 2.) sequenced originating from plasmablasts. The subset contained sequences with isotype IgA1 and a single IgM isotypes. Figure 6. below shows us the phylogenetic tree of the sequences.
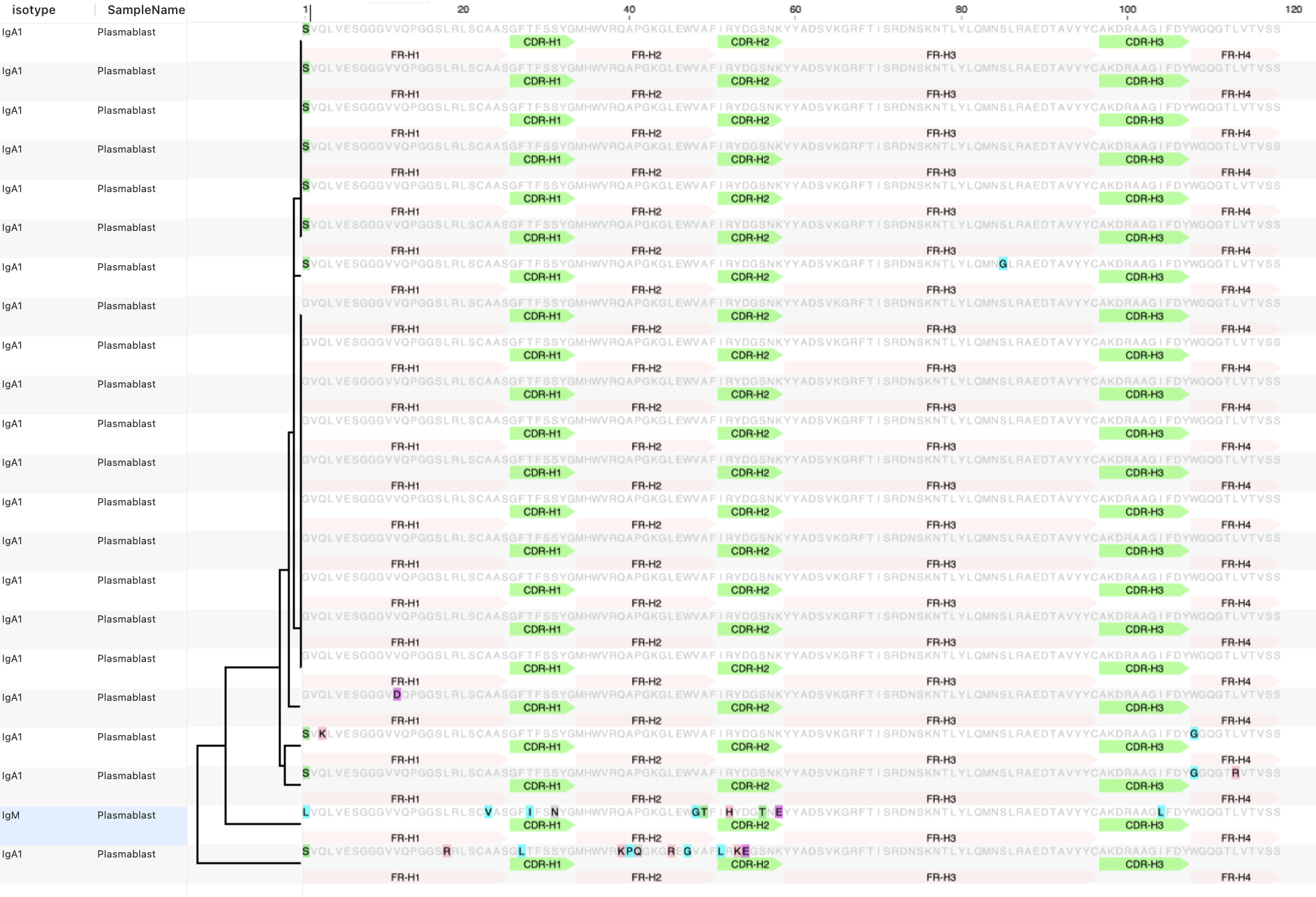
From the sequence alignment above, we can observe a variety of sequences of the class-switched IgA1 isotype and one sequence of isotype IgM. The data used in our analysis is sufficient to describe some relationships between individual sequences and clusters of sequences, but combining the data with transcriptomics data would provide deeper knowledge of class-switching.
For a thorough transcriptomic analysis of single B-cell maturation and class switching, we recommend reading the original article by King et al. (2021).
In conclusion
While combining transcriptomics data and sequence data is required for deep examination of the cell stages and the predictive capability of gene expression, repertoire analysis remains important and provides valuable insights into the development of the antibody repertoire in humans. Having the right tools for analyzing and visualizing the data makes the process faster, easier and repeatable.
For more information on how to import 10x data to PipeBio, see Appendix 1 at the end of this post.
If you’re looking to analyze antibody or BCR repertoire data, you can request to start a trial with us today.
Appendix 1: 10x data on PipeBio.
What data to upload to PipeBio?
We recommend uploading demultiplexed data to PipeBio. Any Cell Ranger pipeline involving vdj will give you an all_contig.fasta file containing contigs and an all_contig_annotations.csv file containing associated barcodes, UMI counts and annotations. To keep all single-cell data connected with your sequences, upload the demultiplexed all_contig-file to PipeBio, followed by the annotations csv-file.
When you upload the csv-file to PipeBio, you only have to select the correct mapping (contig_id <-> name) and you’re good to go.
QC of 10x data on PipeBio
While Cell Ranger already incorporates several QC steps, you also have the option to perform QC on PipeBio by labeling sequences with
- Read counts below threshold
- UMI counts below threshold
- Specific chains.
Running the pipeline will give you additional data with which you can filter and select sequences for further analysis. You can also choose to initially keep all sequences and filter out data later during the analysis.
Appendix 2: 10x Genomics and Cell Ranger
The single-cell technology by 10x Genomics
The single cell library prep and barcoding technology by 10x Genomics allows analyzing up to hundreds of thousands single cells in single runs. By multiplexing cells, that is labeling single cells and then pooling them together, samples can be analyzed all in one batch. The result of all of this is a high-throughput, more affordable method to get multidimensional data on single cells, including
- Full-length V(D)J sequences for paired B-cell or T-cell receptors (BCRs and TCRs)
- Cell surface protein expression
- Antigen specificity
- Gene expression
The unique barcodes in each gel bead-in-emulsion (GEM) allow tying each data point to a single cell. By additionally labeling each transcript within a single cell with a unique molecular identifier (UMI), it is possible to analyze gene expression counts per cell, control for quality based on expression, and correct for amplification bias.
Cell Ranger pipelines and outputs
Cell Ranger – the analysis pipeline software by 10x – has a variety of different processing pipelines for distinct library types (e.g. antibody capture, gene expression) and the outputs vary accordingly. The pipelines in Cell Ranger essentially take as input
- the libraries used and

- the feature reference sheet, containing information about barcode mapping, barcode sequences, how to extract the barcode sequence from the read and and feature type

Depending on the pipeline you’re running (vdj, count or multi), the Cell Ranger will demultiplex and analyze your data on V(D)J repertoires, cell surface proteins, antigen specificity, or gene expression. In brief, what Cell Ranger to demultiplex sequences is
- determine whether the sequences are TCR or BCR
- use barcodes and UMIs to assemble contigs of V(D)J transcripts per cell
- align them to a reference sequence
- output aggregate files that include UMI counts per cell, feature cell matrices and barcoded contigs
After demultiplexing on Cell Ranger, you are then able to take output files and upload them to PipeBio for further analysis.