Leveraging public and proprietary data can help accelerate discovery and shorten the development cycle
Therapeutic antibodies represent one of the fastest growing classes of biologics. Their optimization spans binding affinity, specificity, half-life extension, manufacturability, and immunogenicity risk reduction. Achieving these interrelated objectives requires mining different but interconnected datasets before reliable engineering insights can be drawn.6

Mining antibody data to accelerate discovery and development
Analysis of public antibody data such as WHO INN (International Nonproprietary Name) listings, patent sequences, and next-generation sequencing databases can help to discover and develop better therapeutics antibodies1,6. For example, pairing computational modeling with mining of global clinical pharmacokinetic datasets revealed subtle Fc sequence and glycosylation patterns correlating with improved serum half-life6. Such public data mining illuminates engineering directions worth exploring.
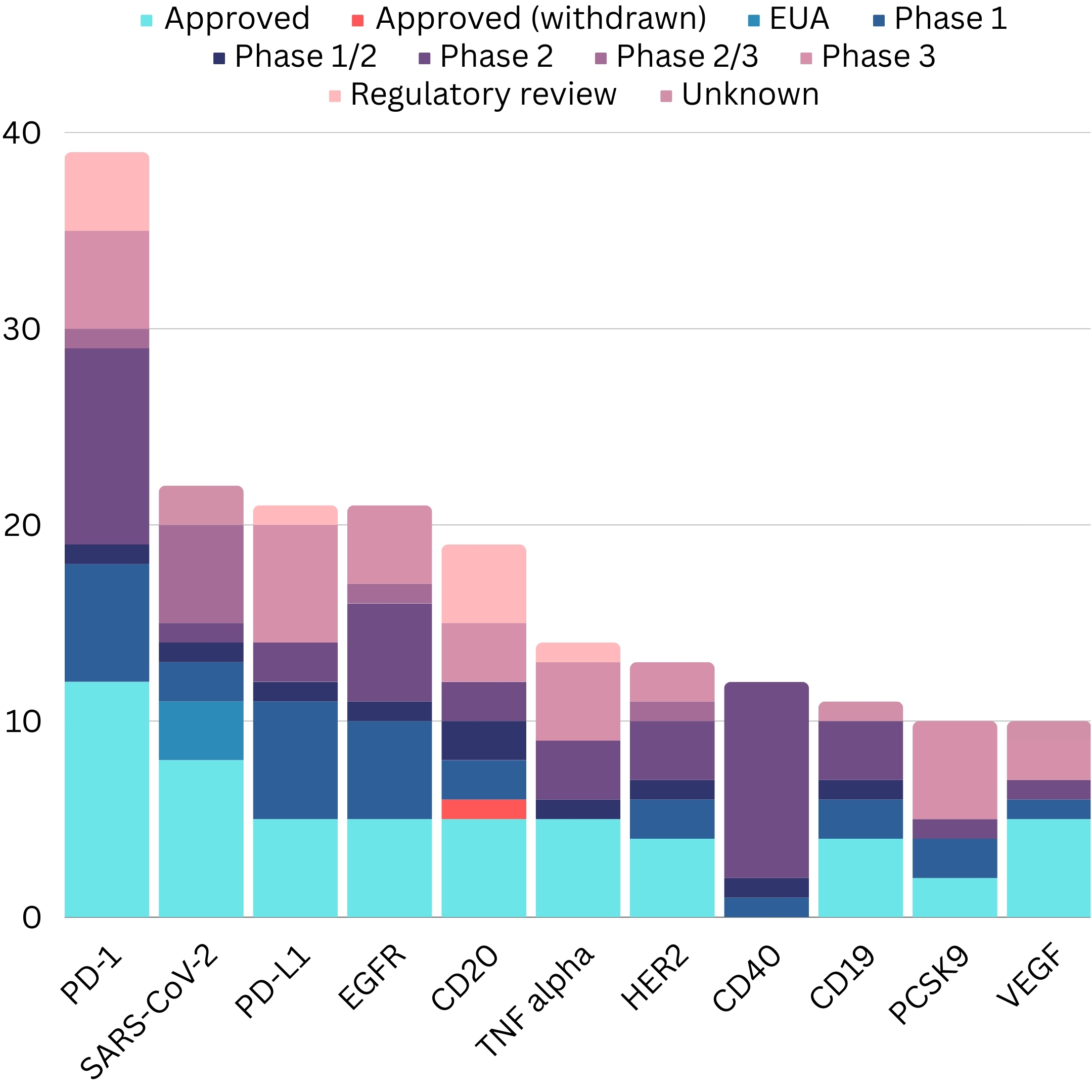
In a recent study, Wilkinson and Hale (2022) collated therapeutic antibody data from public INN registrations, gathering sequence, target, format, highest stage of development, proposed and recommended lists, engineered properties (effector function regulation, half-life, heterodimerization, site specific conjugation, stabilization) and matching patents.
A study from Krawczyk et al. analyzed over 245,000 antibody sequences from 16,526 patent families covering major patent jurisdictions worldwide1. Antibodies comprised 11% of all protein sequences deposited in the USPTO patent database, indicating they make up a substantial proportion of patented biomolecules1. Further analysis revealed that the majority (71.5%) of patents with antibody sequences are explicitly for medicinal applications1. This suggests patents could encapsulate previous engineering efforts to develop clinical antibody candidates.
However, without assaying modifications in antibodies as internal programs and projects, global mining rarely yields directly implementable solutions. Each therapeutic antibody has unique developability and immunogenicity constraints based on its sequence, structure and framework context.2 Still, public data mining provides clues to guide experimentation.
Experimental interrogation of modifications in antibodies
Rational optimization requires experimentally interrogating modifications from global data mining in internal antibodies’ programs and projects. Assaying different variants’ affinity, expression yield, stability, viscosity and immunogenicity in relevant environments generates specific sequence-activity insights2. High-throughput mutational studies systematically evaluate property changes upon introducing modifications, strengthening computational sequence-activity models2.
For example, hit expansion screens leverage one promising lead to drive related antibody optimization2. Synthesizing panels of variants with mutations computationally predicted from global mining to improve developability generates crucial experimental binding data. Comparing variants’ measured biophysical properties and immunogenic potential relative to the original hit facilitates selecting better leads.2
Hit expansion can also be performed through bioinformatic mining of antibody repertoires using tools like PipeBio. This strategy may help discover related, natural antibody clones with better developability profiles than those of previously identified antibody hits in a discovery campaign.
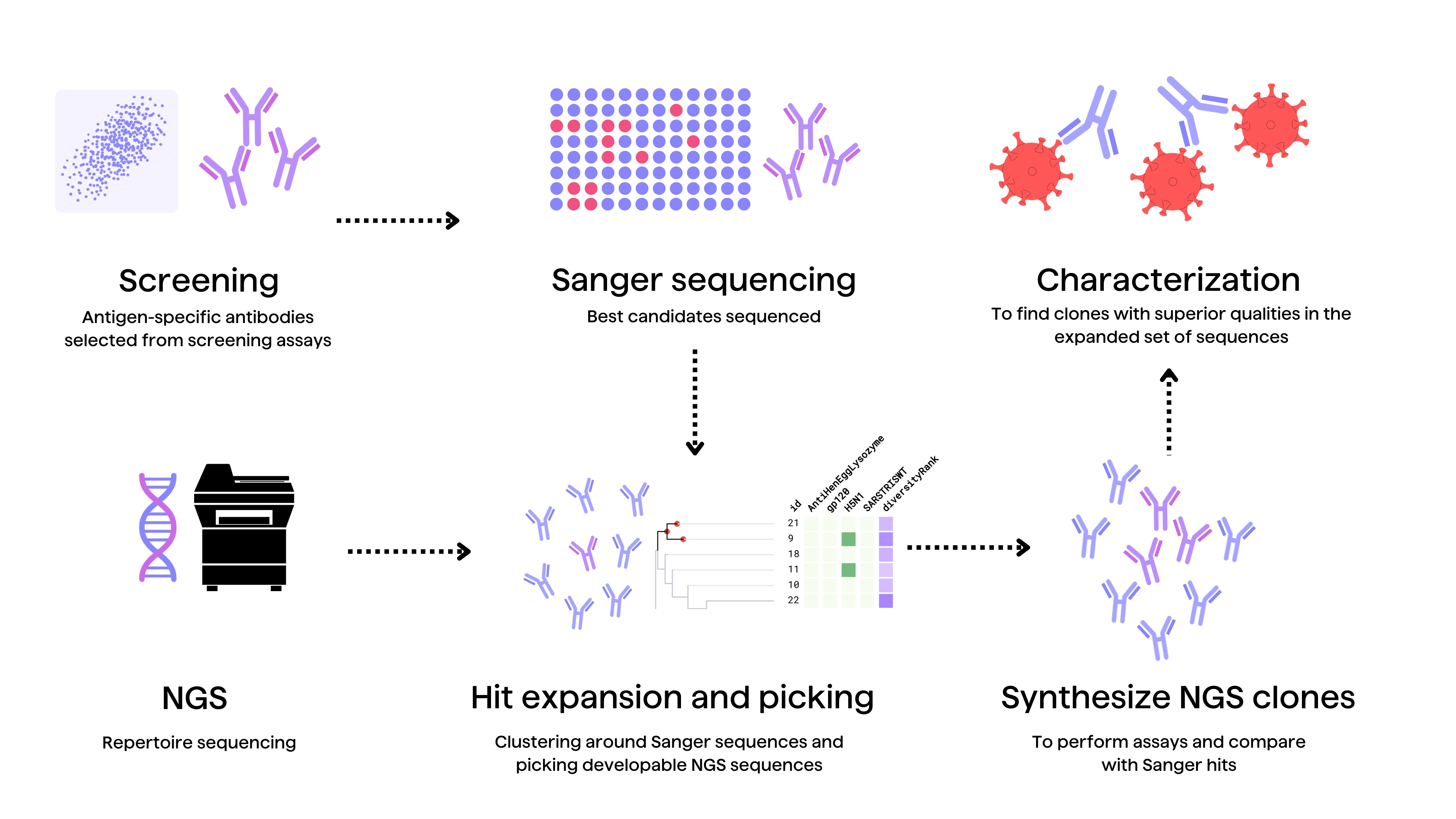
Likewise, experimentally dissecting public mining clues through repertoire mining of internal antibodies databases illuminates paths forward. Here, diverse naïve or synthetic repertoires of antibody sequences related to current leads are expressed and assayed to chart sequence-activity landscapes, uncovering shared motifs that improve developability2. Contrasting naturally occurring and engineered repertoires while assaying their key properties cements sequence-activity relationships.
Mapping Approved Therapeutic Antibodies to Patented Sequences
Critically, almost all (97%) currently approved therapeutic antibody sequences have perfect sequence matches in patents 1. Many patented sequences align with over 90% identity to clinical antibodies 1. While patents aim to legally protect molecules rather than convey scientific data, antibody patent data contains the actual sequences of successful biotherapeutics and variants thereof. The thousands of related antibody sequences in patents likely capture previous engineering optimizations en route to clinical candidates2.
Moreover, immense natural antibody sequence diversity can now be queried using next-generation sequencing (NGS) of immunoglobulin gene repertoires (Ig-seq)3. Over half a billion antibody sequences across diverse immune states and individuals have been collected in the Observed Antibody Space database (OAS)3. Contrasting such naturally occurring antibodies in this database with engineered sequences in patents could help identify modifications that reduce immunogenicity or improve other therapeutic properties4.
Unfortunately, unreliable antibodies can complicate biomedicine research and reproducibility5. Text mining methods can extract statements about antibody specificity issues from the literature to construct knowledge bases alerting users about problematic antibodies5. Integrating such methods with patent mining could help exclude problematic sequences.
Public Data Sources for Obtaining Patent or Public Domain Antibody Data
In case of data mining, access to comprehensive and reliable data is crucial for researchers, clinicians, and industry professionals. Public resources play a pivotal role in enhancing the understanding of therapeutic antibodies in various stages of development, offering insights into their clinical trials, structural details, and overall landscape. Below, you will find 5 examples of sources for obtaining patent or public domain antibody data: Thera-SabDab, PLAbDab, OAS and the Antibody Society and Tabs.
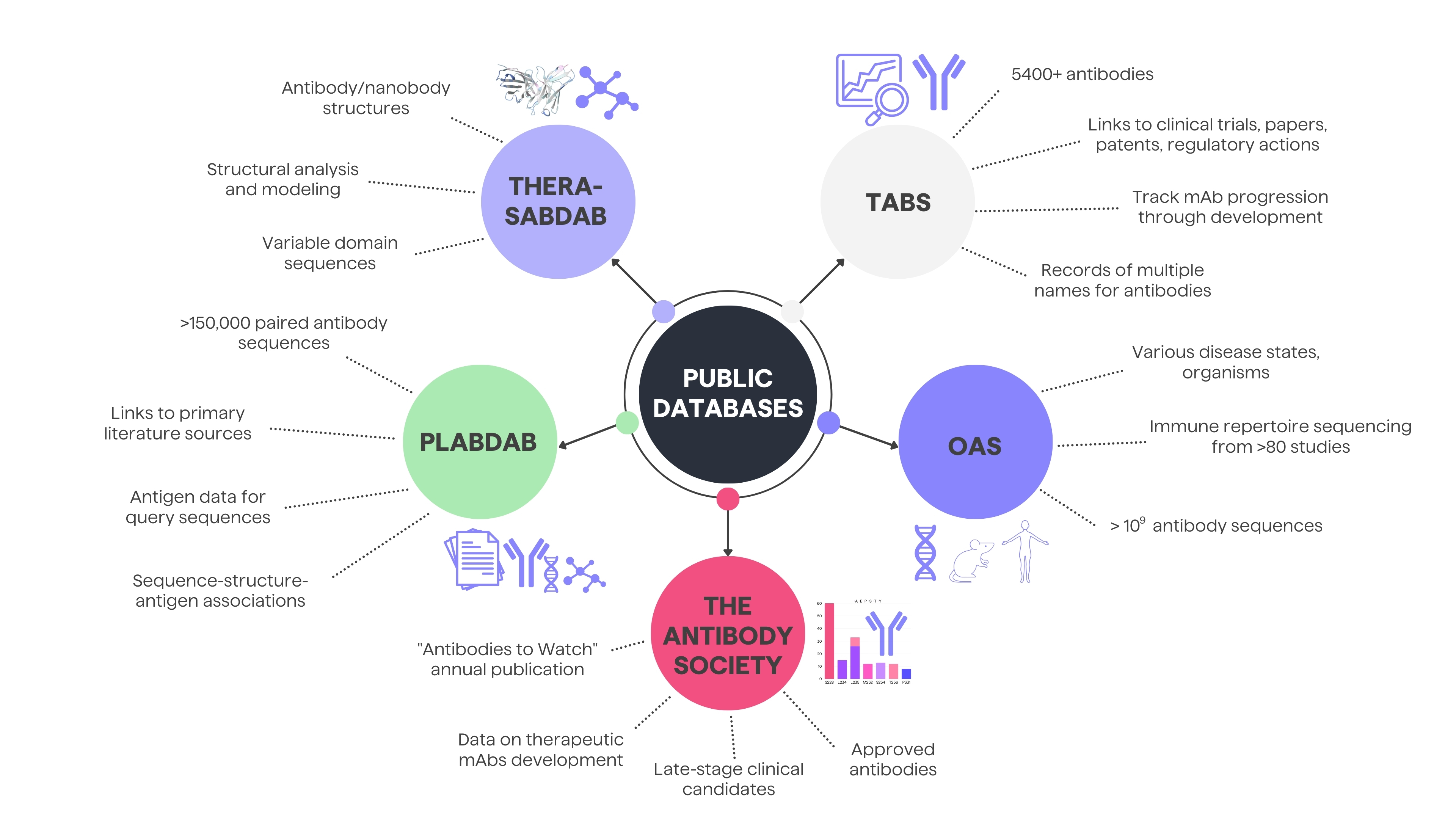
Thera-SabDab
The Therapeutic Structural Antibody Database (Thera-SabDab) specifically maps therapeutic antibody variable domain sequences to known protein structures in the SAbDab structural database. It rapidly detects exact and close sequence matches (99% or 95-98% identity) to facilitate structural analysis and modeling. The curators update Thera-SabDab's sequence-structure mappings weekly alongside SAbDab.8
PLAbDab
The Patent and Literature Antibody Database (PLAbDab) is a self-updating repository containing over 150,000 paired antibody sequences from patents and papers. Each entry links to its primary literature source containing additional functional details. PLAbDab enables annotating query sequences with antigen data from similar entries and analyzing/modifying structural models. Its precomputed structural models enable rapid searching by structure similarity.9
OAS
The Observed Antibody Space database (OAS) collects over one billion annotated antibody sequences from immune repertoire sequencing studies covering various disease states, organisms and individuals. OAS offers downloadable datasets to facilitate data mining and analysis of immune repertoires.10
Antibody Society
The Antibody Society's "Antibodies to Watch" series offers valuable annual updates on monoclonal antibody therapeutics development. These articles document details of late-stage clinical candidates, products under regulatory review, and recently approved antibodies. Comparing the data year-over-year illustrates the remarkable growth - the late-stage pipeline comprised just 26 monoclonal antibodies in 2010 but expanded to 115 by 2022.11
Tabs
The Therapeutic Antibody Database (Tabs) contains detailed information on almost 2000 therapeutic antibodies in development, targeting over 750 antigens across more than 600 companies. Tabs links each antibody entry to clinical trials, papers, patents, regulatory actions, and press releases to enable quick exploration of a candidate's background. It also records the multiple names used for antibodies as they progress through development to ease tracking. Around 60% of antibodies in Tabs are in preclinical stages, offering the full picture beyond just clinical stage assets.7
Integrating Global and Specific Antibody Data Mining
In summary, reliably improving therapeutic antibodies requires an integrated approach pairing global public data mining with focused experimental interrogation of mining-inspired modifications in antibodies in internal programs and projects.2 Computationally integrating these interconnected data types facilitates more rational lead selection and engineering. As both global and specific datasets grow, effectively harnessing data mining algorithms to extract and experimentally validate insights is critical for powering cutting-edge antibody optimization.